Do you find quizzes irresistible? I do.
Here’s a little quiz about working with missing data:
True or False?
1. Imputation is really just making up data to artificially inflate results. It’s better to just drop cases with missing data than to impute.
2. I can just impute the mean for any missing data. It won’t affect results, and improves power.
3. Multiple Imputation is fine for the predictor variables in a statistical model, but not for the response variable.
4. Multiple Imputation is always the best way to deal with missing data.
5. When imputing, it’s important that the imputations be plausible data points.
6. Missing data isn’t really a problem if I’m just doing simple statistics, like chi-squares and t-tests.
7. The worst thing that missing data does is lower sample size and reduce power.
Answers: (more…)
The default approach to dealing with missing data in most statistical software packages is listwise deletion–dropping any case with data missing on any variable involved anywhere in the analysis. It also goes under the names case deletion and complete case analysis.
Although this approach can be really painful (you worked hard to collect those data, only to drop them!), it does work well in some situations. By works well, I mean it fits 3 criteria:
– gives unbiased parameter estimates
– gives accurate (or at least conservative) standard error estimates
– results in adequate power.
But not always. So over the years, a number of ad hoc approaches have been proposed to stop the bloodletting of so much data. Although each solved some problems of listwise deletion, they created others. All three have been discredited in recent years and should NOT be used. They are:
Pairwise Deletion: use the available data for each part of an analysis. This has been shown to result in correlations beyond the 0,1 range and other fun statistical impossibilities.
Mean Imputation: substitute the mean of the observed values for all missing data. There are so many problems, it’s difficult to list them all, but suffice it to say, this technique never meets the above 3 criteria.
Dummy Variable: create a dummy variable that indicates whether a data point is missing, then substitute any arbitrary value for the missing data in the original variable. Use both variables in the analysis. While it does help the loss of power, it usually leads to biased results.
There are a number of good techniques for dealing with missing data, some of which are not hard to use, and which are now available in all major stat software. There is no reason to continue to use ad hoc techniques that create more problems than they solve.
I’m sure I don’t need to explain to you all the problems that occur as a result of missing data. Anyone who has dealt with missing data—that means everyone who has ever worked with real data—knows about the loss of power and sample size, and the potential bias in your data that comes with listwise deletion.
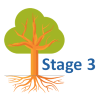
Listwise deletion is the default method for dealing with missing data in most statistical software packages. It simply means excluding from the analysis any cases with data missing on any variables involved in the analysis.
A very simple, and in many ways appealing, method devised to (more…)
SPSS has a nice little feature for adding and averaging variables with missing data that many people don’t know about.
It allows you to add or average variables, while specifying how many are allowed to be missing.
For example, a very common situation is a researcher needs to average the values of the 5 variables on a scale, each of which is measured on the same Likert scale.
There are two ways to do this in SPSS syntax.
Newvar=(X1 + X2 + X3 + X4 + X5)/5 or
Newvar=MEAN(X1,X2, X3, X4, X5).
In the first method, if any of the variables are missing, due to SPSS’s default of listwise deletion, Newvar will also be missing.
In the second method, if any of the variables is missing, it will still calculate the mean. While this seems great at first, the researcher may wish to limit how many of the 5 variables need to be observed in order to calculate the mean. If only one or two variables are present, the mean may not be a reasonable estimate of the mean of all 5 variables.
SPSS has an option for dealing with this situation. Running it the following way will only calculate the mean if any 4 of the 5 variables is observed. If fewer than 4 of the variables are observed, Newvar will be system missing.
Newvar=MEAN.4(X1,X2, X3, X4, X5).
You can specify any number of variables that need to be observed.
(This same distinction holds for the SUM function in SPSS, but the scale changes based on how many are being averaged. A better approach is to calculate the mean, then multiply by 5).
This works the same way in the syntax or in the Transform–>Compute menu dialog.
First Published 12/1/2016;
Updated 7/20/21 to give more detail.