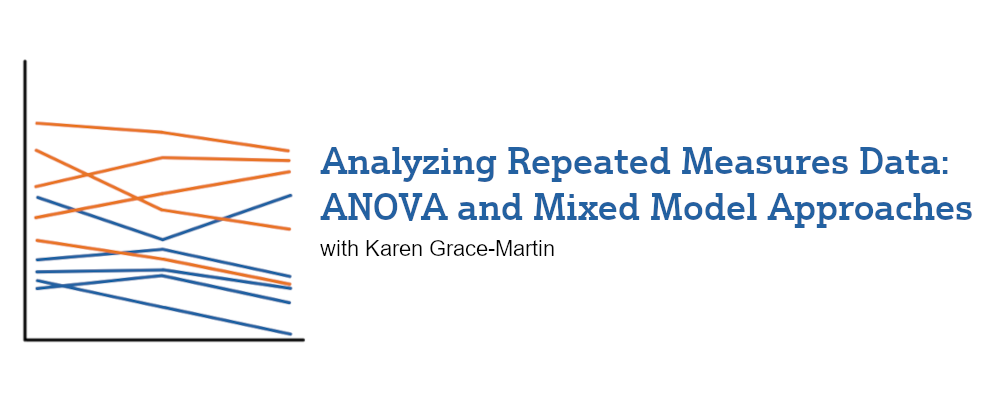
Workshop Enrollment: Analyzing Repeated Measures Data: ANOVA and Mixed Model Approaches
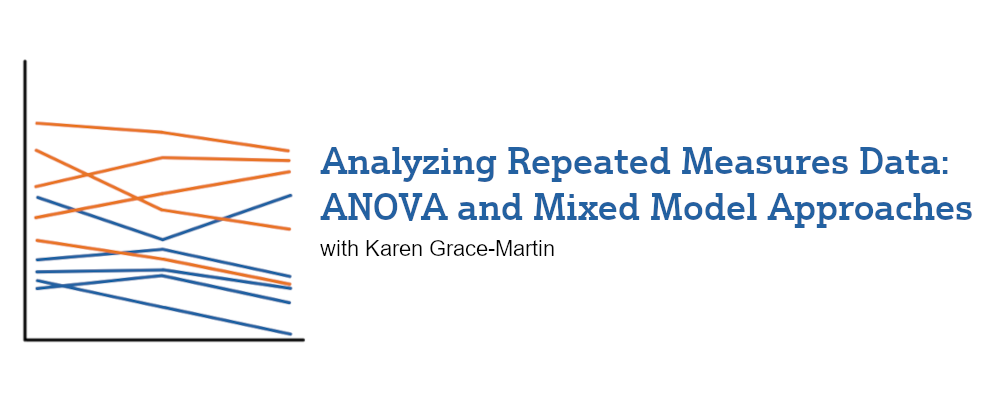
Enrolling Now through September 10, 2024
When it comes to analyzing repeated measures data, there are three main approaches:
- Multivariate GLM (aka, repeated measures ANOVA)
- Marginal Model
- Mixed Model
Each has its own advantages and disadvantages. Depending on what repeated measures designs you’re dealing with, some approaches work better than others.
But do you know when to use which approach?
If you’re like me, you were trained on the GLM (Repeated Measures ANOVA) approach, but its limitations make it far from ideal:
- If even one observation is missing, you lose the whole subject’s data.
- You can’t run post-hoc tests on within-subjects factors (which, unfortunately, is often the whole point!).
- You must have the same number of repeated observations for all subjects… or you have to toss out data.
- You have to assume that all within-subject observations have equal variance/correlations (which isn’t always the case).
- It can’t account for higher-level groupings of subjects into clusters (like kids in the same classroom or patients in the same hospital).
Mixed models solve these problems.
BUT… mixed models are often really hard to learn — especially on your own.
Even if you find a book that covers mixed models, the math is tricky, as they’re written for PhD students in statistics — not researchers.
I struggled with these new, more flexible models for quite a few years. What I needed was a guide that covered repeated measures from the beginning, and fit Linear Mixed Models into what I already understood about mixed ANOVAs.
There simply wasn’t one… so I decided to create a workshop that maps onto common designs that researchers can understand.
Introducing… One of The Analysis Factor’s Most Popular Workshops.
I’m Karen Grace-Martin, workshop instructor.
My goal is that, by the end of the workshop, students will be able to independently run repeated measures, marginal, and mixed models for repeated measures data… and have a solid basis of understanding which approach to choose.
Karen Grace-Martin MA, MA
In this eight-module, online workshop, we’ll cover:
- The difference between fixed, repeated, and random effects
- How to set up your data, run the model, make model-building decisions, and check assumptions and model fit
- The purpose of a covariance structure in different types of models, and which one to choose
- How to interpret and decide when to use random intercepts and random slopes in your model
And in the future, when you do need to look up something in a book on Mixed Modeling… you’ll understand it!
Who Is This Workshop For?
This workshop is for you if you:
- Have heard you should use Mixed Models for your repeated measures data but don’t know how
- Have tried (unsuccessfully) to learn Mixed Models on your own
- Have always run regressions and now are dealing with longitudinal or repeated measures data for the first time
- Are facing a design where regressions won’t work, such as longitudinal or repeated measures data, lots of missing data, or unequal sample sizes
It is NOT for you if you:
- Want to learn Bayesian approaches to Mixed Modeling
- Are new to statistics or regression and ANOVA (You’ll need a solid background in statistics. See below for more details on the course prerequisites)
- Have only run regressions with continuous variables and have never run a repeated measures ANOVA
- Are in search of information on advanced topics like GLMM
- Want a general linear mixed models course and don’t run repeated measures data (this workshop will focus on repeated measures data)
“…I’ve been stumbling through repeated measures analyses with incomplete understanding of the models, and I feel like I’ve had my blinders removed!”
— Hilary Neckles, Research Ecologist, USGS Patuxent Wildlife Research Center, Previous workshop participant —
How Does It Work?
This course is an 8-module online workshop.
All lessons and software demonstrations are pre-recorded. Each week, starting on Thursday morning, we’ll make each module’s materials available. At your convenience, watch the video lessons, watch the video software demonstrations, do the exercises, and review the software syntax of your choice to implement the examples in the lessons.
Have questions? No problem!
On the following Wednesday, you’ll meet with your instructor for a live webinar-based Q&A session where you can get assistance with workshop concepts, deepen your knowledge, and clarify any questions.
All 8 Q&A Sessions Are Recorded for Your Convenience.
Where Do I Access Recordings and Material?
For each of the eight modules, you will have a set of training resources and material.
As a participant of the Analyzing Repeated Measures Data workshop, you can access these for 12 months in our participant-only website.
Data Sets
Real research data sets in Stata, SPSS, SAS, and R formats.
Exercises + Solutions
Try out what you’ve learned in each module to cement the material. Never get stuck with provided syntax and interpretations.
Software Syntax and Demo Videos
Step-by-step videos for Stata, SPSS, SAS, and R. Syntax files available to download so you can save and edit them for your own analysis.
Forum Access
Ask your instructor about workshop content or how to apply it to your own data analysis.
Handouts
Session slides and supplementary material to support your learning.
Q&A Recordings
Can’t make a live Q&A? Submit questions ahead of time and get answers from the recording.
Recorded Lesson Videos
A new recorded lesson released each week so you can watch at your preferred schedule.
Suggested Resources
External videos, articles, or exercises that we recommend to support your learning.
You’ll have access to this site and all the related materials and resources for ONE FULL YEAR. That means you can re-watch sessions and ask additional questions again during that 12-month period.
Often, our students report they understand the material at a deeper level on a second or third pass. You’ll learn more every time, so take advantage of it!
“I was very comfortable with the whole course. [The] descriptions for whom the workshop would be suited — or not — was very helpful…”
— Klaus M. Frei, Previous workshop participant —
What’s Covered in the Workshop?
Module 1: An Overview of Repeated Measures Data
One of the things I’ve found troublesome over the years is that when people say repeated measures, they often mean very different things. There are actually a lot of different designs that can be considered repeated measures.
We specifically will cover:
- Experiments with one or two within-subjects factors, with a possible between subjects design
- Pre-post designs
- Longitudinal studies with pre-, post-, and follow-up measures taken months apart, with some covariates measured only at baseline and some measured at each measurement
- Studies that measured patients five times over two years on an outcome variable only, where only 10% of participants had all five measurements
- Experimental studies where repeated trials were pure replicates
Consequently, we’re going to start by taking away the names which may or may not communicate what we need and examine the structure of the variables and the research questions to think about the real issues:
- What is the role of time?
- Should time be considered continuous or categorical?
- What is the role of baseline measurements?
- Are correlations among a subject’s measurements something of interest or a nuisance?
- Is time confounded with any predictors? Is Subject?
We’ll then go through an overview of the three main approaches so you have the big picture before we delve deeply into each one.
Module 2: The Repeated Measures ANOVA (GLM) Model
This is the “usual” method for running repeated measures data, and probably the one with which you’re most familiar. It does work reasonably well in some situations, and although it doesn’t look like it, there are many similarities between this model and the mixed model.
We’ll discuss the similarities, the differences, and the assumptions this model has that we are able to relax in mixed models.
We’ll go through examples of running a model with this method and interpreting the output so that you have a new understanding of the model you’ve been running for years and its assumptions.
We will then be able to compare two new approaches to this one, so you see where they differ and where they’re really the same.
Module 3: Transitioning to the Mixed Approach
There are many advantages to running repeated measures data using mixed models. But they do require you set up your data differently (in the long, or observation-level format, as opposed to the wide, or person-level format you’re used to).
In this module I’ll give a tutorial on how to restructure the data set from the Wide Format required for repeated measures ANOVA (with one row per subject) to the Long Format, (with one row per observation), as required by mixed model procedures.
I’ll also show you how to graph your data, per person, so you can see what is going on. I have always found this absolutely indispensable in choosing an approach. But you can’t just use a regular means plot or scatter plot, because of the clustering of the data, so once again, I’ll show you just how to do it.
Both the marginal and the mixed model use Maximum Likelihood Estimation, so we’ll do a quick review, then talk at length about measuring model fit.
We’ll still use F tests, but our old friend R-squared disappears as a meaningful measure. In its place comes Deviance, and its Information Criteria buddies.
They’re not so bad once you get used to them, and you’ll see how necessary they become when you are choosing a model later on. Not just which predictors to include, but whether to include various random effects.
Module 4: The Marginal Model and the Repeated Statement
The Marginal Model is not actually a mixed model–there are no random effects, although it is usually run using mixed procedures. (The exception is R, which requires a different command.)
In this session we will explore the Marginal Model (also known as population averaged models) both conceptually and for Repeated Measures Data.
In many repeated measures situations, the marginal model fits the data better than a Mixed Model. It has many of the same advantages as Mixed Models, and is in many ways more intuitive.
It’s a nice bridge because conceptually, it’s very similar to the Repeated Measures ANOVA you’re used to. It too takes into account the correlation among each subject’s observations. But it’s more flexible than the Repeated Measures ANOVA because it allows those correlations to be unique (goodbye, Sphericity assumption! Hello, post-hoc tests on within subject factors!).
The result is more choices, and often better model fit.
Module 5: The Mixed Model: Random Intercept Models
If we stopped before this module, you would already have a whole new set of tools that greatly expand the types of analyses you can do, and do well.
But being able to run mixed models will exponentially expand the types of research questions you can answer.
For repeated measures data, there are two types of mixed models you’ll use–random intercept models and individual growth curve models.
Random intercept models take care of the correlation among a subject’s responses as do marginal models, but they do it in a different way. Instead of measuring correlations, they actually measure how much responses vary across individuals. If one individual generally has high responses, and another always low, the random intercept measures and accounts for that. It literally redefines the residuals.
We can then measure how much of the variation in the outcome is due to the covariates, the treatment conditions, or the subjects themselves. So here you will learn how to use statistics like variance components and Intraclass Correlation to understand the variation in responses over time, conditions, or individuals.
This module introduces the simplest linear mixed model in depth before going on to random slope models.
Module 6: The Mixed Model: Individual Growth Curve (Random Slope) Model
Individual growth curve models go a step further–they allow each subject to have a different growth trajectory over time–or over any other covariate that changes over time.
In many, many designs, the random intercept model is enough to take care of similarities in individuals’ responses.
But when the real research questions are how factors affect growth (or decline) over time, growth models answer the question directly.
We’ll talk about what individual growth curve models are, how to run them, when to use them, and when not to.
We’ll also explore issues like improving interpretation through centering, when missing data causes no problems at all, and and how the models differ when time is measured as continuous or categorical.
We’ll also introduce covariance structures for random effects.
Module 7: Model Refinements, Extensions, and Assumptions
Now that you’ve got a good understanding of the model, what it measures, and what the various statistics mean, we’re going to talk about ways to improve it.
We’ll start by checking whether or not the newly-defined residuals meet assumptions of normality and independence. If they don’t, we can account for it by refining the residual covariance structure with a repeated statement. Nice, eh?
Then we’ll go over a few common extensions– three-level models for when individuals are clustered into classrooms or companies or some other grouping and Random Curvature models, which allow growth to vary from a linear trajectory.
Module 8: Building and Choosing a Model
Okay, now that you’ve learned how to create and refine linear mixed models, how do you choose?
There are many, many choices along the way–the type of model, which random effects to include, which covariance structures–before you even get to choosing which predictors to put into the model.
There are two main approaches to take to make all these decisions–you either start with the most complex model and simplify, or start with the simplest and add complexity.
Here we go through two very different examples start to finish to bring together everything you’ve learned. You will learn the exact steps to take to build the model and choose the best one.
Dates and Times
The Workshop has 8 modules. Each module includes: video lessons, software demonstrations and syntax files, exercises and answers, and a live Q&A session.
Workshop Orientation (Optional):
Wednesday, September 11 at 2pm – 2:30pm (EST)
Q&A Session Dates: Wednesdays, 2pm – 3pm (US ET)
Q&A Session Dates
Q&A 1 – September 18
Q&A 2 – September 25
Q&A 3 – October 2
Q&A 4 – October 9
**Break Week** (October 14 – 19)
Q&A 5 – October 23
Q&A 6 – October 30
Q&A 7 – November 6
Q&A 8 – November 13
Remember, everything is recorded and available for you to watch at your convenience, should you be unable to attend a live session.
Also, you have access to all the workshop materials for a full 12 months from your enrollment date.
Sorry, enrollment for this workshop is not currently open.
Please contact us if you have any questions
or get on the waiting list to be notified when it's open again.
About Your Instructor
Hi, I’m Karen Grace-Martin, your workshop instructor.
As president and founder of The Analysis Factor, I’ve been supporting researchers like you through their statistical planning, analysis, and interpretation since 1997.
My goal is to help you improve your statistical literacy so you can bring your important research results into the light with confidence.
Karen Grace-Martin MA, MA
“The instructor was delightfully pleasant, knowledgeable, slow-paced, non-judgmental, and responsive… Thank you for letting those of us overwhelmed by other time constraints time to absorb it all.”
— Previous workshop participant —
Prerequisites
So what kind of background in statistics do you need?
This course starts out at a moderate level, but we do move into more advanced material. No, you don’t need a PhD in statistics… we know you’re a researcher, not a statistician.
We’re assuming:
- You haven’t taken linear algebra
- You don’t want to see things derived
- You’re here because you want to see these concepts explained in English, not formulas
That being said, you should be fairly statistics savvy. In other words, this shouldn’t be your first exposure to running linear models. You should be familiar with:
- Centering
- Least-squares estimation
- Dummy variables
- Interactions
- Residuals
- Variance
- Regression coefficients
You’ll get the most from this workshop if you have:
- MINIMUM two statistics classes, one of which MUST include linear models — multiple regression or Analysis of Variance (ANOVA)
- Real experience doing some sort of linear modeling (familiarity with the GLM procedure will be very beneficial)
- SPSS users: It will help if you’re familiar with SPSS Syntax. (Some parts using menus will be demonstrated in class, but at this level of modeling, you must have control of the details at the level that syntax allows.)
If you have questions about whether you’re ready, just email us. We’ll give you our honest opinion. We want you to succeed!
Your Satisfaction Is Guaranteed
As with all of our programs, your satisfaction is guaranteed. Your registration fee is fully refundable for any reason up to the close of enrollment, no questions asked. Once the refund period ends and the workshop begins, we want you to be satisfied. If you participate fully in a workshop – watch, read, and try out what is included – and find you are not satisfied, we will give you a full refund. Just notify us within 90 days of purchasing the program.
FAQs
Q: I haven’t taken a statistics course in years. Will I be able to keep up?
A: As long as you have the suggested prerequisites (a strong understanding of linear regression), you should be fine.
However, you WILL need to spend some time each week, both going over the material and also “getting your hands dirty” with some data. Expect to spend 4-5 hours per week reviewing the material and doing the exercises, and re-running class examples on your own.
And if you have questions during the workshop, make sure to attend the Q&A sessions and/or post on the Workshop Center to clarify anything you’re not clear on.
You know how this goes: The more time you put in, the deeper your understanding.
Q: What’s your refund policy?
A: Your registration fee is fully refundable for any reason up to the close of enrollment, no questions asked. Once the refund period ends and the workshop begins, we want you to be satisfied. If you participate fully in a workshop – watch, read, and try out what is included – and find you are not satisfied, we will give you a full refund. Just notify us within 90 days of purchasing the program.
Q: Do you offer student discounts?
A: Yes, we do! Current students with a valid ID can register for up to 20% off the standard rate. Click here to receive our student discount on all our workshops: Student Discount List
Q: Things are very busy at work right now, and I’m afraid I won’t have time to keep up. How much time per week does this really take?
A: We’ve worked hard to make sure people can take a workshop in the midst of their normal work life. For longer workshops, we build in a week off partway through the course.
Each module’s lesson contains 90 minutes to 2 hours of videos. You may also want to view our software demonstration videos. We include those for four software programs. You’ll obviously need more time if you watch more than one.
Each week, we have a one-hour Q&A session. You don’t have to attend; we make those available so you can have any questions answered or listen in on others’ questions.
We provide exercises, answers and data sets, so you can practice what you’ve learned each week and get help in the Q&As. These will take you a few hours each week, maybe up to 3 or so. The exercises aren’t required — you’re not graded. But we’ve gotten feedback that they’re very helpful, as it allows you to practice and figure out what you did and didn’t understand.
In other words, each week, there are approximately 2 hours of instruction, one hour of Q&A, and about 2-3 hours of practice time. So if you have a crazy hectic week during the workshop, you have catch up time. But If you have a crazy hectic month, you may be better off waiting as you won’t really have a chance to catch up.
One reminder: You have access to the workshop website, all materials, and a place to ask questions for a year. So if you get behind, you aren’t missing out. You can catch up on your schedule.
Q: I teach/work/sleep during this time and cannot attend live. Is there any way to register and access the webinar sessions later?
A: Yes! We have participants from many time zones with many different work schedules. In order to support our diverse student base, all material – including the workshop sessions and Q&As — are recorded and made available to students within 48 hours. They’re screenshot video files, so you’ll hear the instructor talking and see his screen, just as live participants do. You can even submit questions for written answers any time between sessions. Many students take the entire workshop in this manner, never attending a session live.
You can also download and keep these videos forever, so if you need to refresh your memory at any point in the future, it’s yours to review.
Q: I’m outside the U.S. Can I still participate?
A: Yes. We have participants in our workshops from many different countries. You will want a fast internet connection and either a computer speaker or a telephone if you plan to attend live.
Q: Can I pay with Paypal?
A: Yes. When you check out, you can pay directly using our system or paying with Paypal. However, the three-payment option is not available using PayPal.
Q: Can I join the Q&A webinars from my tablet or phone?
A: Yes. You can join all webinar sessions from your Apple or Android device by downloading a free GoToMeeting app.
Q: This really isn’t a good time for me. Will you be offering this workshop again soon?
A: We don’t have a set schedule for running each workshop. We try to schedule many of our workshop every 18 months, and our more popular workshops may be offered more often. We are sometimes unable to re-run a workshop if the instructor becomes unavailable so there are no guarantees that any workshop will be run again.
If you think you’ll need the material in the next half-year or so, I would suggest registering now. You’ll get all the materials to download and review at your convenience, and you can attend again for free any time in the next 12 months.
Have additional questions? We’re here to help! Just email us at support@theanalysisfactor.com.