The Intraclass Correlation Coefficient, or ICC, can be very useful in many statistical situations, but especially so in Linear Mixed Models.
Linear Mixed Models are used when there is some sort of clustering in the data.
Two common examples of clustered data include:
- individuals sampled within sites (hospitals, companies, community centers, schools, etc.). The site is the cluster.
- repeated measures or longitudinal data where you collect multiple observations from the same individual. The individual is the cluster in which multiple observations are (more…)
Graphing predicted values from a regression model or means from an ANOVA makes interpretation of results much easier.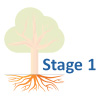
Every statistical software will graph predicted values for you. But the more complicated your model, the harder it can be to get the graph you want in the format you want.
Excel isn’t all that useful for estimating the statistics, but it has some very nice features that are useful for doing data analysis, one of which is graphing.
In this webinar, I will demonstrate how to calculate predicted means from a linear and a logistic regression model, then graph them. It will be particularly useful to you if you don’t have a very clear sense of where those predicted values come from.
Note: This training is an exclusive benefit to members of the Statistically Speaking Membership Program and part of the Stat’s Amore Trainings Series. Each Stat’s Amore Training is approximately 90 minutes long.
About the Instructor
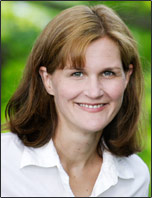
Karen Grace-Martin helps statistics practitioners gain an intuitive understanding of how statistics is applied to real data in research studies.
She has guided and trained researchers through their statistical analysis for over 15 years as a statistical consultant at Cornell University and through The Analysis Factor. She has master’s degrees in both applied statistics and social psychology and is an expert in SPSS and SAS.
Not a Member Yet?
It’s never too early to set yourself up for successful analysis with support and training from expert statisticians.
Just head over and sign up for Statistically Speaking.
You'll get access to this training webinar, 130+ other stats trainings, a pathway to work through the trainings that you need — plus the expert guidance you need to build statistical skill with live Q&A sessions and an ask-a-mentor forum.
Hierarchical regression is a very common approach to model building that allows you to see the incremental contribution to a model of sets of predictor variables.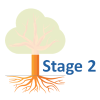
Popular for linear regression in many fields, the approach can be used in any type of regression model — logistic regression, linear mixed models, or even ANOVA.
In this webinar, we’ll go over the concepts and steps, and we’ll look at how it can be useful in different contexts.
Note: This training is an exclusive benefit to members of the Statistically Speaking Membership Program and part of the Stat’s Amore Trainings Series. Each Stat’s Amore Training is approximately 90 minutes long.
About the Instructor
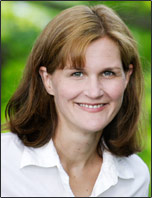
Karen Grace-Martin helps statistics practitioners gain an intuitive understanding of how statistics is applied to real data in research studies.
She has guided and trained researchers through their statistical analysis for over 15 years as a statistical consultant at Cornell University and through The Analysis Factor. She has master’s degrees in both applied statistics and social psychology and is an expert in SPSS and SAS.
Not a Member Yet?
It’s never too early to set yourself up for successful analysis with support and training from expert statisticians.
Just head over and sign up for Statistically Speaking.
You'll get access to this training webinar, 130+ other stats trainings, a pathway to work through the trainings that you need — plus the expert guidance you need to build statistical skill with live Q&A sessions and an ask-a-mentor forum.
Have you ever been told you need to run a mixed (aka: multilevel) model and been thrown off by all the new vocabulary?
It happened to me when I first started my statistical consulting job, oh so many years ago. I had learned mixed models in an ANOVA class, so I had a pretty good grasp on many of the concepts.
But when I started my job, SAS had just recently come out with Proc Mixed, and it was the first time I had to actually implement a true multilevel model. I was out of school, so I had to figure it out on the job.
And even with my background, I had a pretty steep learning curve to get to a point where it made sense. Sure, I was able to figure out the steps, but there are some pretty tricky situations and complicated designs out there.
To implement it well, you need a good understanding of the big picture, and how the small parts fit into it. (more…)
One area in statistics where I see conflicting advice is how to analyze pre-post data. I’ve seen this myself in consulting. A few years ago, I received a call from a distressed client. Let’s call her Nancy.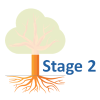
Nancy had asked for advice about how to run a repeated measures analysis. The advisor told Nancy that actually, a repeated measures analysis was inappropriate for her data.
Nancy was sure repeated measures was appropriate. This advice led her to fear that she had grossly misunderstood a very basic tenet in her statistical training.
The Study Design
Nancy had measured a response variable at two time points for two groups. The intervention group received a treatment and a control group did not. Participants were randomly assigned to one of the two groups.
The researcher measured each participant before and after the intervention.
Analyzing the Pre-Post Data
Nancy was sure that this was a classic repeated measures experiment. It has (more…)
If you’ve ever worked with multilevel models, you know that they are an extension of linear models. For a researcher learning them, this is both good and bad news.
The good side is that many of the concepts, calculations, and results are familiar. The down side of the extension is that everything is more complicated in multilevel models.
This includes power and sample size calculations. (more…)