Need to dummy code in a Cox regression model?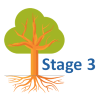
Interpret interactions in a logistic regression?
Add a quadratic term to a multilevel model?
This is where statistical analysis starts to feel really hard. You’re combining two difficult issues into one.
You’re dealing with both a complicated modeling technique at Stage 3 (survival analysis, logistic regression, multilevel modeling) and tricky effects in the model (dummy coding, interactions, and quadratic terms).
The only way to figure it all out in a situation like that is to break it down into parts. (more…)
There are three main ways you can approach analyzing repeated measures data, assuming the dependent variable is measured
continuously: repeated measures ANOVA, Mixed Models, and Marginal Models. Let’s take a look at how the three approaches differ and some of their advantages and disadvantages.
For a few, very specific designs, you can get the exact same results from all three approaches. This makes it difficult to figure out what each one is doing, and how to apply them to OTHER designs.
For the sake of the current discussion, I will define repeated measures data as repeated measurements of the same outcome variable on the same individual. The individual is often a person, but could just as easily be a plant, animal, colony, company, etc. For simplicity, I’ll use “individual.” (more…)
Can I use SPSS MIXED models for (a) ordinal logistic regression, and (b) multi-nomial logistic regression?
Every once in a while I get emailed a question that I think others will find helpful. This is definitely one of them.
My answer:
No.
(And by the way, this is all true in SAS as well. I’ll include the SAS versions in parentheses). (more…)
This free, one-hour webinar is part of our regular Craft of Statistical Analysis series. In it, we will introduce and demonstrate two of the core concepts of mixed modeling—the random intercept and the random slope.
Most scientific fields now recognize the extraordinary usefulness of mixed models, but they’re a tough nut to crack for someone who didn’t receive training in their methodology.
But it turns out that mixed models are actually an extension of linear models. If you have a good foundation in linear models, the extension to mixed models is more of a step than a leap. (Okay, a large step, but still).
You’ll learn what random intercepts and slopes mean, what they do, and how to decide if one or both are needed. It’s the first step in understanding mixed modeling.
This webinar has already taken place. You can gain free access to a video recording of the webinar by completing the form below.
Here’s what participants said about the webinar:
“Thank you. I was also impressed with the way of explaining and the selection of example chosen to explain the theory.”
– Joanna Konieczna-Salamatin
“Teriffic job! I learned a lot. Thanks. Way to reduce a challenging topic to managable bite-size pieces. The graphical representations of the models helped me understand the random slope and random intercept terminology in a way I never got before.”
– Rob Baer
“I found it a great example and clear explanation, an hour is much better spent watching this than reading through a text book as an intro to this form of modeling.”
– Matt Cooper
“It was my first webinar and I was apprehensive with my lack of experience with the tecnology but it was really easy, user friendly, and definitely an experience to be repeated! Thank you!”
– Vanda Roque
” Just terrific. Clear, at the right level for me, extremely helpful.”
– Amy D’Andrade
“The seminar was well presented. The speaking was clear and easily undersood. The presentation was paced well. I found many of the questions and answers at the end to be *very* useful.”
– Andrew McLachlan
This page is out-of-date.
Please go to the newer version of the page: Random Intercept and Random Slope Models COSA webinar.
There are two ways to run a repeated measures analysis.The traditional way is to treat it as a multivariate test–each response is considered a separate variable.The other way is to it as a mixed model.While the multivariate approach is easy to run and quite intuitive, there are a number of advantages to running a repeated measures analysis as a mixed model.
First I will explain the difference between the approaches, then briefly describe some of the advantages of using the mixed models approach. (more…)