My poor colleague was pulling her hair out in frustration today.
You know when you’re trying to do something quickly, and it’s supposed to be easy, only it’s not? And you try every solution you can think of and it still doesn’t work?
And even in the great age of the Internet, which is supposed to know all the things you don’t, you still can’t find the answer anywhere?
Cue hair-pulling.
Here’s what happened: She was trying to import an Excel spreadsheet into SAS, and it didn’t work.
Instead she got:
(more…)
Graphing predicted values from a regression model or means from an ANOVA makes interpretation of results much easier.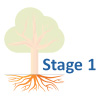
Every statistical software will graph predicted values for you. But the more complicated your model, the harder it can be to get the graph you want in the format you want.
Excel isn’t all that useful for estimating the statistics, but it has some very nice features that are useful for doing data analysis, one of which is graphing.
In this webinar, I will demonstrate how to calculate predicted means from a linear and a logistic regression model, then graph them. It will be particularly useful to you if you don’t have a very clear sense of where those predicted values come from.
Note: This training is an exclusive benefit to members of the Statistically Speaking Membership Program and part of the Stat’s Amore Trainings Series. Each Stat’s Amore Training is approximately 90 minutes long.
About the Instructor
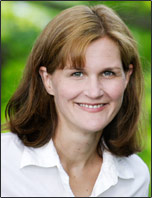
Karen Grace-Martin helps statistics practitioners gain an intuitive understanding of how statistics is applied to real data in research studies.
She has guided and trained researchers through their statistical analysis for over 15 years as a statistical consultant at Cornell University and through The Analysis Factor. She has master’s degrees in both applied statistics and social psychology and is an expert in SPSS and SAS.
Not a Member Yet?
It’s never too early to set yourself up for successful analysis with support and training from expert statisticians.
Just head over and sign up for Statistically Speaking.
You'll get access to this training webinar, 130+ other stats trainings, a pathway to work through the trainings that you need — plus the expert guidance you need to build statistical skill with live Q&A sessions and an ask-a-mentor forum.
This year I hired a Quickbooks consultant to bring my bookkeeping up from the stone age. (I had been using Excel).
She had asked for some documents with detailed data, and I tried to send her something else as a shortcut. I thought it was detailed enough. It wasn’t, so she just fudged it. The bottom line was all correct, but the data that put it together was all wrong.
I hit the roof.Internally, only—I realized it was my own fault for not giving her the info she needed. She did a fabulous job.
But I could not leave the data fudged, even if it all added up to the right amount, and already reconciled. I had to go in and spend hours fixing it. Truthfully, I was a bit of a compulsive nut about it.
And then I had to ask myself why I was so uptight—if accountants think the details aren’t important, why do I? Statisticians are all about approximations and accountants are exact, right?
As it turns out, not so much.
But I realized I’ve had 20 years of training about the importance of data integrity. Sure, the results might be inexact, the analysis, the estimates, the conclusions. But not the data. The data must be clean.
Sparkling, if possible.
In research, it’s okay if the bottom line is an approximation. Because we’re never really measuring the whole population. And we can’t always measure precisely what we want to measure. But in the long run, it all averages out.
But only if the measurements we do have are as accurate as they possibly can be.