When you draw a graph- either a bar chart, a scatter plot, or even a pie chart, you have the choice of a broad range of colors that you can use. R, for example, has 657 different colors from aliceblue to yellowgreen. SAS has 13 shades of orange, 33 shades of blue, and 47 shades of green. They even have different shades of black.
You have a wealth of colors, but you can’t use all of them in the same graph. The ideal number of colors is 2.
(more…)
Do you ever wish your data analysis project were a little more organized?
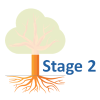
(more…)
Tell me if you can relate to this: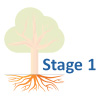
You love your field of study, you enjoy asking the big questions and discovering answers. But, when it comes to data analysis and statistics you get a little bogged down. You might even feel a bit lost sometimes.
And that is hard to admit.
Because after all, you are supposed to be the expert. Right?
Learning Statistics is Hard but We Make it a Lot Easier
The thing is, statistics is a field unto itself. But as a researcher, you need to be adept with statistics even though you may only have very basic training in this field. In essence, you learned statistics — a new language — out of context. You had no real immersion experience to practice this language. You had few opportunities to apply the strange new terms and concepts as you learned them.
At the Analysis Factor, we understand the pain of learning and doing statistics. We have been in the trenches with hundreds of researchers like you across many fields of study. Everyone is struggling to grow their statistical, data analysis, and software skills.
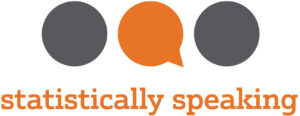
In Statistically Speaking we support and guide you as you learn — every step of the way. We know where to start, where to go next, and next, and next.
We know that your field and research question(s) determine the type of data and complexity of statistical analyses you will choose. And we know that everyone shows up in a different place, and needs different things to help them get where they need to go.
So we have created a treasure trove of resources on hundreds of topics — from data cleaning and research design to logistic regression and structural equation modeling.
And to keep it all about you, we have created a customizable learning platform, one where you make a plan for your own unique journey. We have crafted a series of comprehensive Maps, curated guides on essential topics at each Stage of mastery, offering you a structured pathway through the maze of statistical knowledge.
You create the plan you need, and choose the maps you need to do your research.
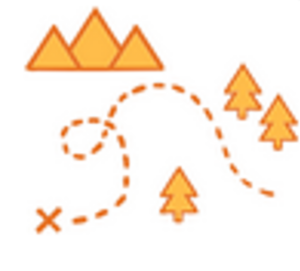
Maps
At The Analysis Factor, we classify the statistical content and skills into 4 Stages to help you decide where to begin your learning journey. In Statistically Speaking, the Maps are categorized into these Stages.
Here are just a few examples:
Stage 1: Fundamentals
-
Preparing Data: Understanding the fundamental steps in data preparation, from cleaning and transforming to structuring datasets for analysis.
-
Bivariate Statistics: Grasping the basics of relationships between two variables, laying the groundwork for more complex analyses.
Stage 2: Linear Models
-
Graphing: Learning visualization techniques to represent data and derive meaningful insights.
-
Introduction to Regression: Unraveling the fundamentals of regression analysis, a cornerstone of statistical modeling.
-
Interpreting Results: Developing the skill to interpret statistical results and draw valid conclusions from analyses.
Stage 3: Extensions of Linear Models
-
Count Models: Exploring specialized models for count data analysis, understanding their application and nuances.
-
Logistic Regression: Diving into binary outcome analysis, understanding probabilities, and logistic models.
-
Factor Analysis: Delving into multivariate analysis, understanding latent variables and their relationships.
Stage 4: Advanced Models
-
GLMM: Embracing the complexity of generalized linear mixed models, integrating fixed and random effects.
-
SEM: Venturing into structural equation modeling, exploring complex relationships among variables.
-
Survival Analysis: Understanding time-to-event data, its application in various fields, and survival modeling techniques.
By mapping out the key content and skills you want to learn at each Stage, you’ll gain a clearer understanding of the vast statistical landscape and feel empowered to take on the learning journey ahead.
So, what are you waiting for? Members, head on over to explore the Maps in Statistically Speaking.
And if you are not yet a member, you can sign-up for our waitlist to join Statistically Speaking. We would love to meet you, learn about your research, and help you get started on your statistical learning adventure.
In statistical practice, there are many situations where best practices are clear. There are many, though, where they aren’t. The granddaddy of these practices is adjusting p-values when you make multiple comparisons. There are good reasons to do it and good reasons not to. It depends on the situation.
At the heart of the issue is a concept called Family-wise Error Rate (FWER). FWER is the probability that
(more…)
The classic way to compare means in analysis of variance is examining pairwise differences in means after an F test.. It’s great for many problems, but sometimes your research question isn’t about pairwise differences.
Pairwise differences are not ideal if your research question is if, like the Sesame Street song, one of these groups is not like the others. Perhaps, you are trying to assure that groups conform to a common standard. Analysis of Means (ANOM) helps in all these settings. (more…)
One of the hardest steps in any project is learning to ask the right research question!
(more…)