Sample size estimates are one of those data analysis tasks that look straightforward, but once you try to do one, make you want to bang your head against the computer in frustration. Or, maybe
that’s just me.
Regardless of how they make you feel, they are super important to do for your study before you collect the data.
(more…)
A research study rarely involves just one single statistical test. And multiple testing can result in more statistically significant findings just by chance.
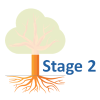
After all, with the typical Type I error rate of 5% used in most tests, we are allowing ourselves to “get lucky” 1 in 20 times for each test. When you figure out the probability of Type I error across all the tests, that probability skyrockets.
(more…)
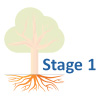
Statistics is, to a large extent, a science of comparison. You are trying to test whether one group is bigger, faster, or smarter than another.
You do this by setting up a null hypothesis that your two groups have equal means or proportions and an alternative hypothesis that one group is “better” than the other. The test has interesting results only when the data you collect ends up rejecting the null hypothesis.
But there are times when the interesting research question you’re asking is not about whether one group is better than the other, but whether the two groups are equivalent.
(more…)
Transformations don’t always help, but when they do, they can improve your linear regression model in several ways simultaneously.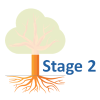
They can help you better meet the linear regression assumptions of normality and homoscedascity (i.e., equal variances). They also can help avoid some of the artifacts caused by boundary limits in your dependent variable — and sometimes even remove a difficult-to-interpret interaction.
(more…)
Interpreting regression coefficients can be tricky, especially when the model has interactions or categorical predictors (or worse – both).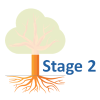
But there is a secret weapon that can help you make sense of your regression results: marginal means.
They’re not the same as descriptive stats. They aren’t usually included by default in our output. And they sometimes go by the name LS or Least-Square means.
And they’re your new best friend.
So what are these mysterious, helpful creatures?
What do they tell us, really? And how can we use them?
(more…)
Ah, logarithms. They were frustrating enough back in high school. (If you even got that far in high school math.)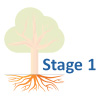
And they haven’t improved with age, now that you can barely remember what you learned in high school.
And yet… they show up so often in data analysis.
If you don’t quite remember what they are and how they work, they can make the statistical methods that use them seem that much more obtuse.
So we’re going to take away that fog of confusion about exponents and logs and how they work. (more…)