One of the most difficult steps in calculating sample size estimates is determining the smallest scientifically meaningful effect size.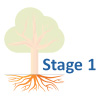
Here’s the logic:
The power of every significance test is based on four things: the alpha level, the size of the effect, the amount of variation in the data, and the sample size.
You will measure the effect size in question differently, depending on which statistical test you’re performing. It could be a mean difference, a difference in proportions, a correlation, regression slope, odds ratio, etc.
When you’re planning a study and estimating the sample size needed for (more…)
Meta-analysis allows us to synthesize the results of separate studies. The goal is to assess the mean effect size and also heterogeneity – how much the effect size varies across studies. (more…)
Effect size statistics are required by most journals and committees these days — for good reason.
They communicate just how big the effects are in your statistical results — something p-values can’t do.
But they’re only useful if you can choose the most appropriate one and if you can interpret it.
This can be hard in even simple statistical tests. But once you get into complicated models, it’s a whole new story. (more…)
Last week I had the pleasure of teaching a webinar on Interpreting Regression Coefficients. We walked through the output of a somewhat tricky regression model—it included two dummy-coded categorical variables, a covariate, and a few interactions.
As always seems to happen, our audience asked an amazing number of great questions. (Seriously, I’ve had multiple guest instructors compliment me on our audience and their thoughtful questions.)
We had so many that although I spent about 40 minutes answering (more…)
Meta-analysis is the quantitative pooling of data from multiple studies. Meta-analysis done well has many strengths, including statistical power,
precision in effect size estimates, and providing a summary of individual studies.
But not all meta-analyses are done well. The three threats to the validity of a meta-analytic finding are heterogeneity of study results, publication bias, and poor individual study quality.
(more…)