Imputation as an approach to missing data has been around for decades.
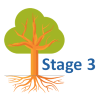
You probably learned about mean imputation in methods classes, only to be told to never do it for a variety of very good reasons. Mean imputation, in which each missing value is replaced, or imputed, with the mean of observed values of that variable, is not the only type of imputation, however. (more…)
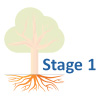
Missing data causes a lot of problems in data analysis. Unfortunately, some of the “solutions” for missing data cause more problems than they solve.
(more…)
There are a number of simplistic methods available for tackling the problem of missing data. Unfortunately there is a very high likelihood that each of these simplistic methods introduces bias into our model results.
Multiple imputation is considered to be the superior method of working with missing data. It eliminates the bias introduced by the simplistic methods in many missing data situations.
(more…)
A data set can contain indicator (dummy) variables, categorical variables and/or both. Initially, it all depends upon how the data is coded as to which variable type it is.
For example, a categorical variable like marital status could be coded in the data set as a single variable with 5 values: (more…)
One important consideration in choosing a missing data approach is the missing data mechanism—different approaches have different assumptions about the mechanism.
Each of the three mechanisms describes one possible relationship between the propensity of data to be missing and values of the data, both missing and observed.
The Missing Data Mechanisms
Missing Completely at Random, MCAR, means there is no relationship between (more…)
Two methods for dealing with missing data, vast improvements over traditional approaches, have become available in mainstream statistical software in the last few years.
Both of the methods discussed here require that the data are missing at random–not related to the missing values. If this assumption holds, resulting estimates (i.e., regression coefficients and standard errors) will be unbiased with no loss of power.
The first method is Multiple Imputation (MI). Just like the old-fashioned imputation (more…)