the craft of statistical analysis free webinars
Approaches to Missing Data: The Good, the Bad, and the Unthinkable
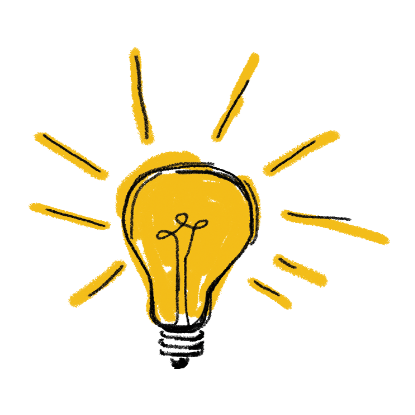
You’ve probably heard about many different approaches to dealing with missing data, and you’ve probably gotten different opinions about which one you should use. learn more
Answers to Questions from the Approaches to Missing Data Webinar
articles at the analysis factor
approaches to dealing with missing data
Multiple Imputation: 5 Recent Findings that Change How to Use It
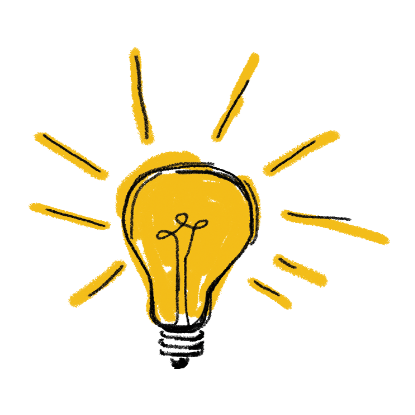
Missing Data, and multiple imputation specifically, is one area of statistics that is changing rapidly. Research is still ongoing, and each year new findings on best practices and new techniques in software appear. learn more
When Listwise Deletion Works for Missing Data
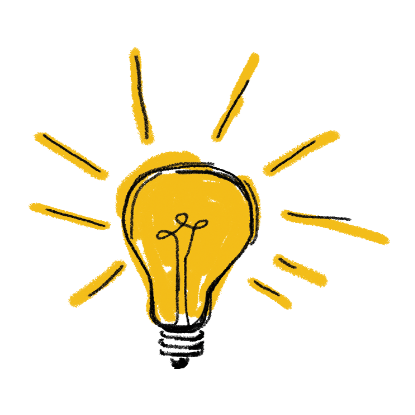
You may have never heard of listwise deletion for missing data, but you’ve probably used it. Listwise deletion means that any individual in a data set is deleted from an analysis if they’re missing data on any variable in the analysis. It’s the default in most software packages. learn more
Missing Data Mechanisms: A Primer
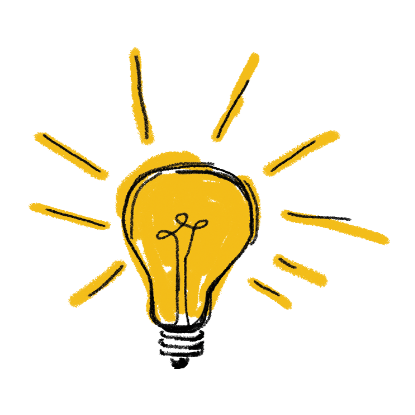
Missing data are a widespread problem, as most researchers can attest. Whether data are from surveys, experiments, or secondary sources, missing data abound. But what’s the impact on the results of statistical analysis? That depends on two things: the mechanism that led the data to be missing and the way in which the data analyst deals with it. learn more
Quiz Yourself about Missing Data
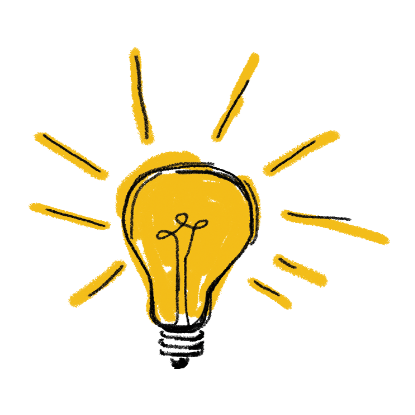
Do you find quizzes irresistible? I do. Here’s a little quiz about working with missing data. learn more
Answers to the Missing Data Quiz
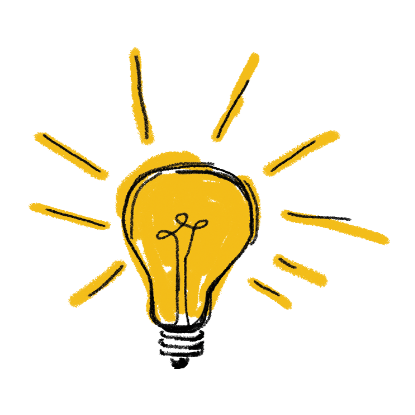
In my last post, I gave a little quiz about missing data. This post has the answers. If you want to try it yourself before you see the answers, go here. (It’s a short quiz, but if you’re like me, you find testing yourself irresistible). learn more
3 Ad-hoc Missing Data Approaches that You Should Never Use
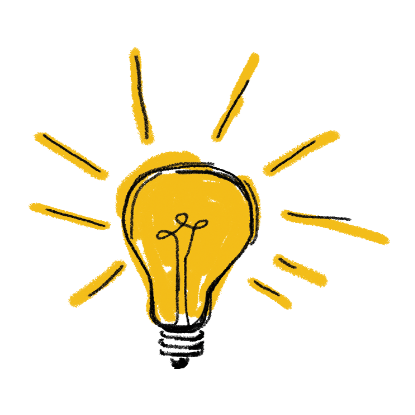
The default approach to dealing with missing data in most statistical software packages is listwise deletion–dropping any case with data missing on any variable involved anywhere in the analysis. It also goes under the names case deletion and complete case analysis. learn more
Multiple Imputation of Categorical Variables
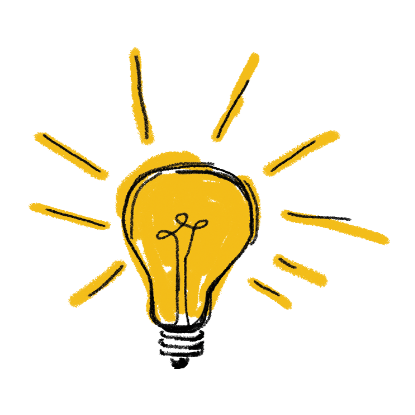
Most Multiple Imputation methods assume multivariate normality, so a common question is how to impute missing values from categorical variables. Paul Allison, one of my favorite authors of statistical information for researchers, did a study that showed that the most common method actually gives worse results that listwise deletion. learn more
Missing Data: Criteria for Choosing an Effective Approach
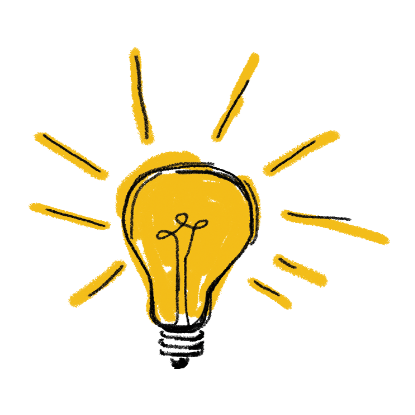
In choosing an approach to missing data, there are a number of things to consider. But you need to keep in mind what you’re aiming for before you can even consider which approach to take. There are three criteria we’re aiming for with any missing data technique. learn more
EM Imputation and Missing Data: Is Mean Imputation Really so Terrible?
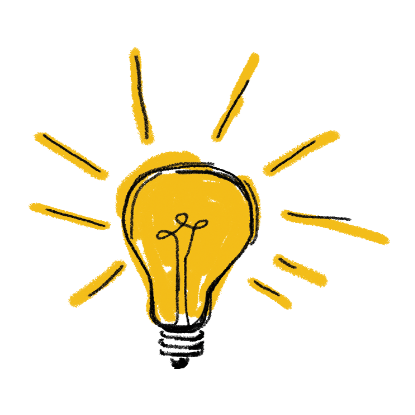
I’m sure I don’t need to explain to you all the problems that occur as a result of missing data. Anyone who has dealt with missing data — that means everyone who has ever worked with real data — knows about the loss of power and sample size, and the potential bias in your data that comes with listwise deletion. learn more
Seven Ways to Make Up Data: Common Methods to Imputing Missing Data
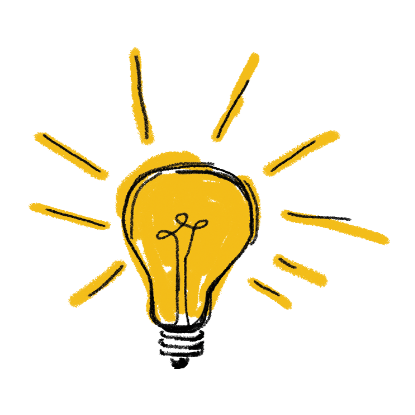
There are many ways to approach missing data. The most common, I believe, is to ignore it. But making no choice means that your statistical software is choosing for you. Most of the time, your software is choosing listwise deletion. Listwise deletion may or may not be a bad choice, depending on why and how much data are missing. learn more
Missing Data: Two Big Problems with Mean Imputation
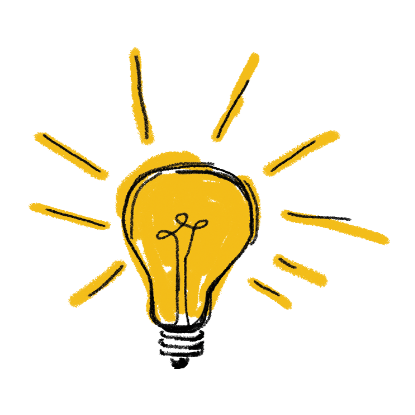
Mean imputation: So simple. And yet, so dangerous. Perhaps that’s a bit dramatic, but mean imputation (also called mean substitution) really ought to be a last resort. It’s a popular solution to missing data, despite its drawbacks. Mainly because it’s easy. It can be really painful to lose a large part of the sample you so carefully collected, only to have little power. learn more
Multiple Imputation in a Nutshell
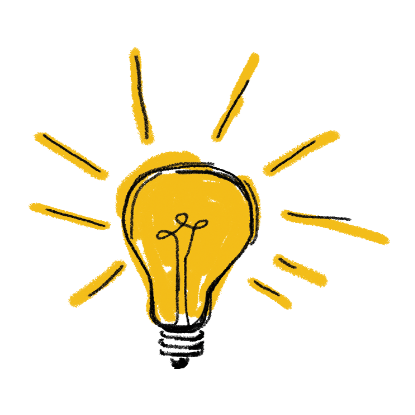
Imputation as an approach to missing data has been around for decades. You probably learned about mean imputation in methods classes, only to be told to never do it for a variety of very good reasons. Mean imputation is not the only type of imputation, however. learn more
Two Recommended Solutions for Missing Data: Multiple Imputation and Maximum Likelihood
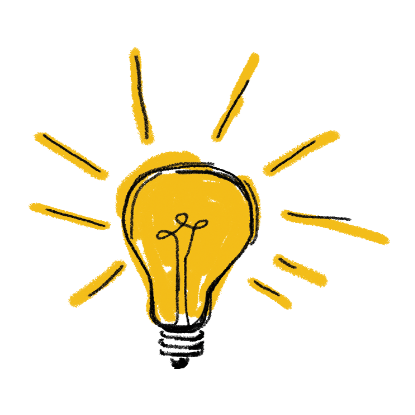
Two methods for dealing with missing data, vast improvements over traditional approaches, have become available in mainstream statistical software in the last few years. Both of the methods discussed here require that the data are missing at random–not related to the missing values. learn more
software
Computing Cronbach’s Alpha in SPSS with Missing Data
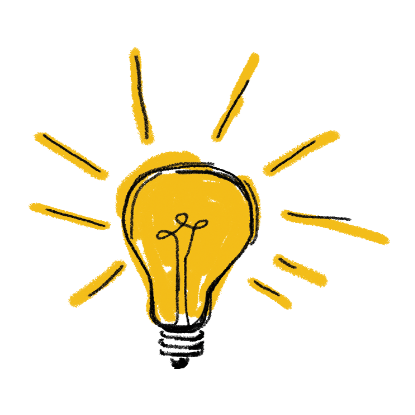
In RELIABILITY, the SPSS command for running a Cronbach’s alpha, the only options for Missing Data are to include or exclude User-Defined missing data. And by exclude, they mean listwise deletion. learn more
New Version Released of Amelia II: A Program for Missing Data
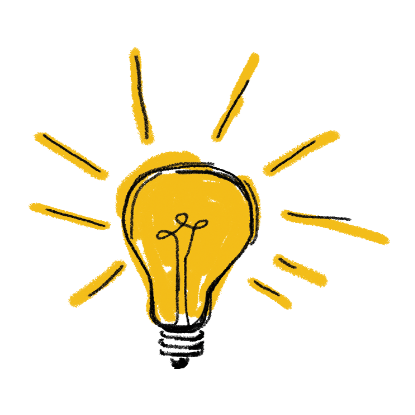
A new version of Amelia II, a free package for multiple imputation, has been released Amelia II is available in two versions. One is part of R, and the other, AmeliaView, is a GUI package that does not require any knowledge of the R programming language. They both use the same underlying algorithms and both require having R installed. learn more
Averaging and Adding Variables with Missing Data in SPSS
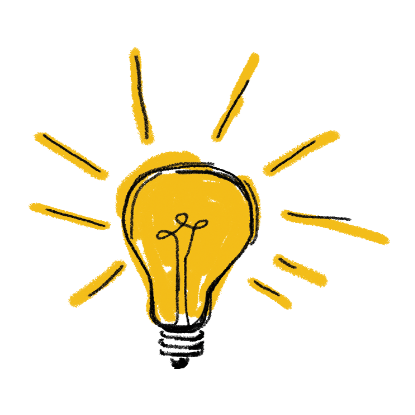
SPSS has a nice little feature for adding and averaging variables with missing data that many people don’t know about. It allows you to add or average variables, while specifying how many are allowed to be missing. learn more
missing data in the context of data analysis
The Steps for Running any Statistical Model
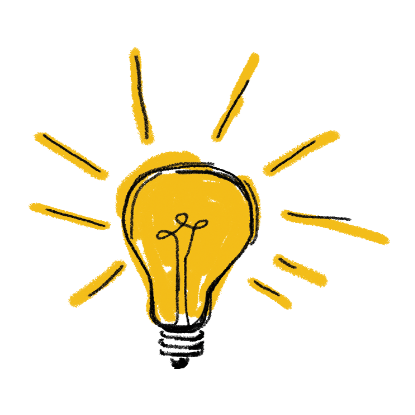
No matter what statistical model you’re running, you need to go through the same steps. The order and the specifics of how you do each step will differ depending on the data and the type of model you use. learn more
Five Advantages of Running Repeated Measures ANOVA as a Mixed Model
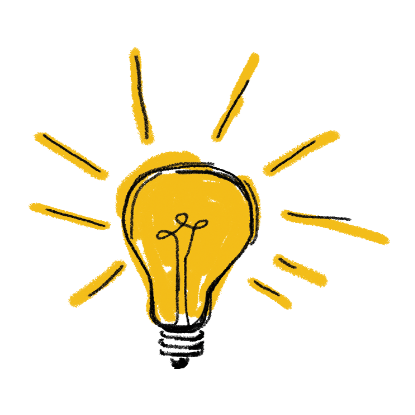
There are two ways to run a repeated measures analysis. The traditional way is to treat it as a multivariate test–each response is considered a separate variable. The other way is to it as a mixed model. While the multivariate approach is easy to run and quite intuitive, there are a number of advantages to running a repeated measures analysis as a mixed model. learn more
recommended books
Missing Data
by Paul Allison
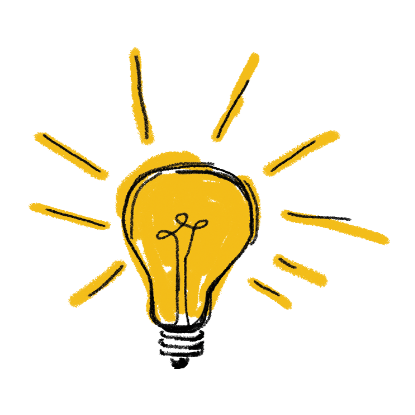
Very reader-friendly. One of “the little green Sage books.” This is an excellent overview, covers much of what a data analyst needs to know, and very accessible. This is the book to start with. And very reasonably priced. learn more
Analysis of Incomplete Multivariate Data
by Joseph Schafer
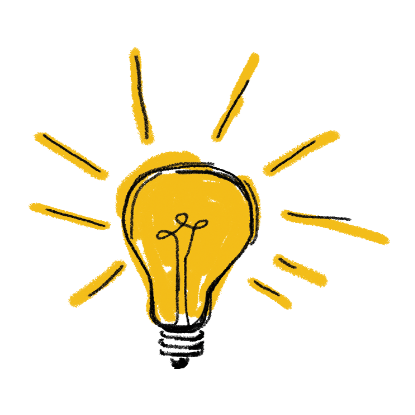
This book is the basis for Joe’s series of multiple imputation programs in S-Plus. It is somewhat more readable than Little and Rubin (below). learn more
Statistical Analysis with Missing Data, Second Edition
by Roderick Little and Donald Rubin
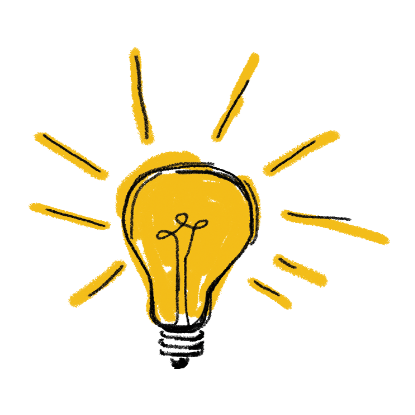
This is the Missing Data Bible. It can get pretty technical at times, but can be worth working through. learn more
journal articles and other resources
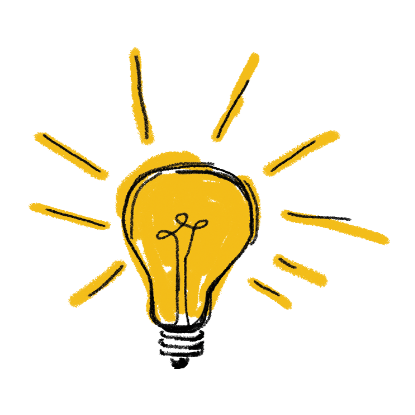
Allison, P.D. (2000). Multiple Imputation for Missing Data: A Cautionary Tale. Sociological Methods and Research, 28, 301-309.
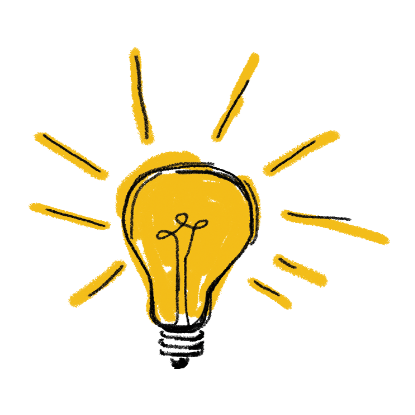
Allison, P.D. (1987) Estimation of linear models with incomplete data. In C. Clogg [Ed.] Sociological Methodology. San Francisco: Jossey Bass, 71-103.
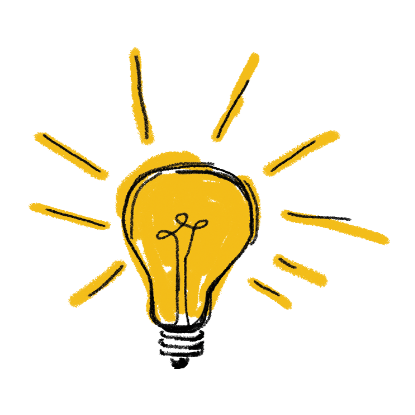
Graham, J. W., & Hofer, S. M. (2000). Mulitple imputation in multivariate research. In T. D. Little, K. U. Schnabel, & J. Baumert, (Eds.), Modeling longitudinal and multiple-group data: Practical issues, applied approaches, and specific examples. Hillsdale, NJ: Erlbaum.This chapter is a very user-friendly description of the use of Joe Schafer’s NORM program, with an illustrative empirical example. (Also see Schafer & Olsen — below — for the same kind of information).
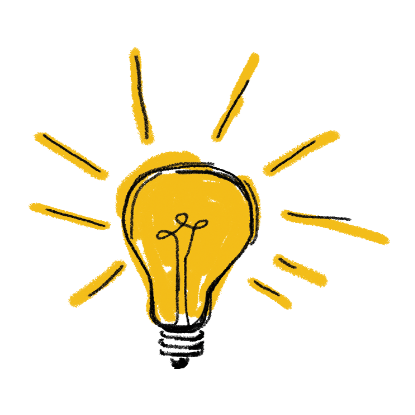
Graham, J. W., Hofer, S.M., Donaldson, S.I., MacKinnon, D.P., & Schafer, J.L. (1997). Analysis with missing data in prevention research. In K. Bryant, M. Windle, & S. West (Eds.), The science of prevention: methodological advances from alcohol and substance abuse research. (pp. 325-366). Washington, D.C.: American Psychological Association.In the context of an empirical example, this chapter discusses, and illustrates the pros and cons of four acceptable, and readily available methods: (a) raw data maximum likelihood with Amos; (b) multiple imputation with NORM; (c) multiple imputation with EMCOV; and (d) EM algorithm (with EMCOV) and bootstrap. We show how the following “old” methods fall very short of desiriable treatment of missing data (listwise deletion, pairwise deletion, mean substitution).
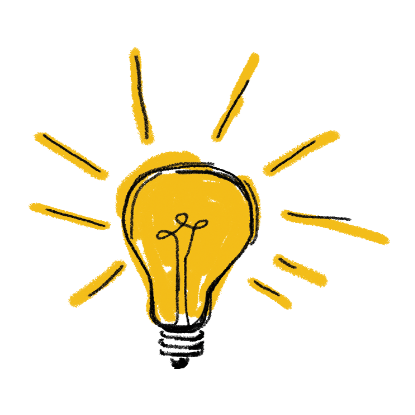
Graham, J.W. & Donaldson, S.I. (1993) Evaluating interventions with differential attrition: The importance of nonresponse mechanisms and use of follow-up data. Journal of Applied Psychology, 78, 119-128
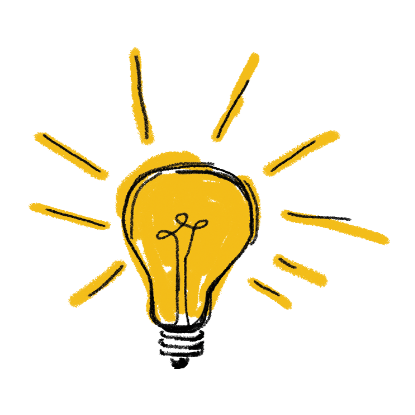
Horton, N. J. & Lipsitz, S.R. (2001). Multiple Imputation in Practice: comparison of Software Packages for Regression Models with Missing Variables. The American Statistician, 55, 244-254.
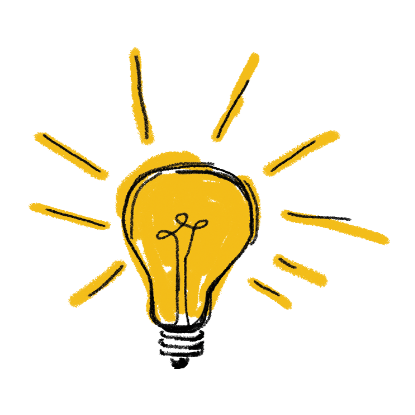
Horton, N.J. & Kleinman, K.P. (2007). Much Ado about Nothing: A Comparison of Missing Data Methods and Software to Fit Incomplete Data Regression Models. The American Statistician, 61, 79-90.
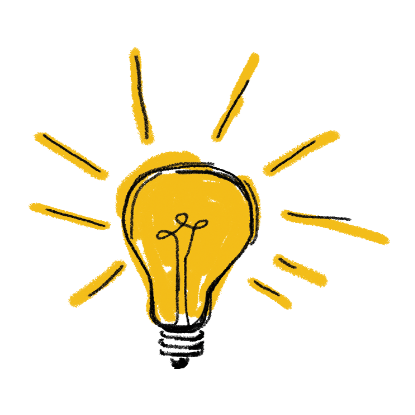
Muthén, B.O., Kaplan, D., & Hollis, M. (1987). On structural equation modeling with data that are not missing completely at random. Psychometrika, 52, 431-462
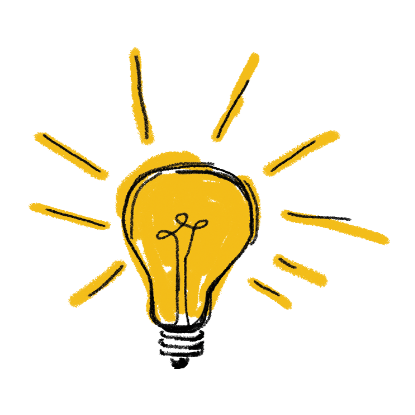
Schafer, J.L. & Graham, J.W. (2002). Missing Data: Our View of the State of the Art. Psychological Methods, 7, 147-177.
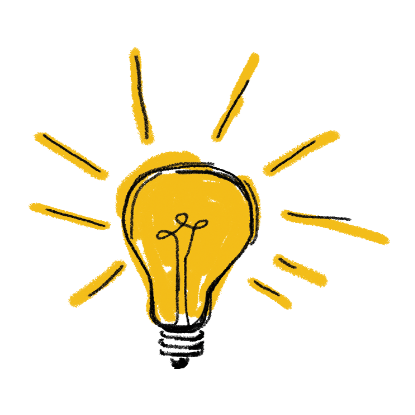
Wothke, W. (2000) Longitudinal and multi-group modeling with missing data. (Adobe pdf format) In T.D. Little, K.U. Schnabel, and J. Baumert [Eds.] Modeling longitudinal and multiple group data: Practical issues, applied approaches and specific examples. Mahwah, NJ: Lawrence Erlbaum Associates. (Reproduced with permission).
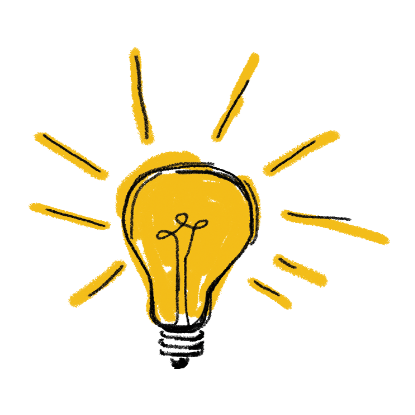
Schafer, J.L. & Graham, J.W. (2002). Missing Data: Our View of the State of the Art. Psychological Methods, 7, 147-177. This is a very well-written overview of the new approaches to dealing with missing data. Joe Schaefer is one of the top statististicians doing research on Missing data techniques and John Graham runs the statistical consulting center at Penn State. Together they explain these new techniques in understandable ways.