When you put a continuous predictor into a linear regression model, you assume it has a constant relationship with the dependent variable along the predictor’s range. But how can you be certain? What is the best way to measure this?
And most important, what should you do if it clearly isn’t the case?
Let’s explore a few options for capturing a non-linear relationship between X and Y within a linear regression (yes, really). (more…)
Need to dummy code in a Cox regression model?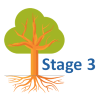
Interpret interactions in a logistic regression?
Add a quadratic term to a multilevel model?
This is where statistical analysis starts to feel really hard. You’re combining two difficult issues into one.
You’re dealing with both a complicated modeling technique at Stage 3 (survival analysis, logistic regression, multilevel modeling) and tricky effects in the model (dummy coding, interactions, and quadratic terms).
The only way to figure it all out in a situation like that is to break it down into parts. (more…)
A polynomial term–a quadratic (squared) or cubic (cubed) term turns a linear regression model into a curve. But because it is X that is squared or cubed, not the Beta coefficient, it still qualifies as a linear model. This makes it a nice, straightforward way to model curves without having to model complicated non-linear models.
But how do you know if you need one–when a linear model isn’t the best model? (more…)