When analyzing longitudinal data, do you use regression or structural equation based approaches? There are many types of longitudinal data and different approaches to analyzing them. Two popular approaches are a regression based approach and a structural equation modeling based approach.
(more…)
The objective for quasi-experimental designs is to establish cause and effect relationships between the dependent and independent variables. However, they have one big challenge in achieving this objective: lack of an established control group.
(more…)
There are not a lot of statistical methods designed just to analyze ordinal variables.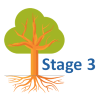
But that doesn’t mean that you’re stuck with few options. There are more than you’d think.
Some are better than others, but it depends on the situation and research questions.
Here are five options when your dependent variable is ordinal.
(more…)
When you learned analysis of variance (ANOVA), it’s likely that the emphasis was on the ANOVA table, with its Sums of Squares and F tests, followed by a post-hoc test. But ANOVA is quite flexible in how it can compare means. A large part of that flexibility comes from its ability to perform many types of statistical contrast.
That F test can tell you if there is evidence your categories are different from each other, which is a start. It is, however, only a start. Once you know at least some categories’ means are different, your next question is “How are they different?” This is what a statistical contrast can tell you.
What is a Statistical Contrast?
A statistical contrast is a comparison of a combination of the means of two or more categories. In practice, they are usually performed as a follow up to the ANOVA F test. Most statistical programs include contrasts as an optional part of ANOVA analysis. (more…)
Exact and randomization tests are simple from a conceptual level and need fewer assumptions than traditional parametric tests. They do require substantial computing power, but nothing that can’t be handled by the computer you have today. (more…)
If you’ve ever run a one-way analysis of variance (ANOVA), you’re familiar with post-hoc tests. The ANOVA omnibus test only tells you whether any groups differ in their means. But if you want to explore which specific group mean is different from which, you need to follow up with a post-hoc test.
(more…)