In the last post, we examined how to use the same sample when running a set of regression models with different predictors.
Adding a predictor with missing data causes cases that had been included in previous models to be dropped from the new model.
Using different samples in different models can lead to very different conclusions when interpreting results.
Let’s look at how to investigate the effect of the missing data on the regression models in Stata.
The coefficient for the variable “frequent religious attendance” was negative 58 in model 3 and then rose to a positive 6 in model 4 when income was included. Results (more…)
You may have never heard of listwise deletion for missing data, but you’ve probably used it without knowing it. Even when it doesn’t work well. 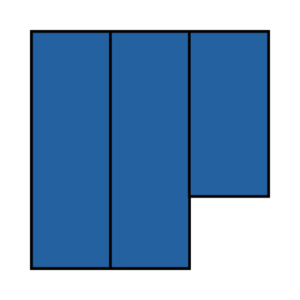
Listwise deletion means that any case in a data set is deleted from an analysis if they’re missing data on any variable in the analysis.
It’s the default in most statistical software packages.
Although its simplicity is a major advantage, it causes big problems in many missing data situations.
But…not always. If you happen to have one of the uncommon missing data situations in which (more…)
One important consideration in choosing a missing data approach is the missing data mechanism—different approaches have different assumptions about the mechanism.
Each of the three mechanisms describes one possible relationship between the propensity of data to be missing and values of the data, both missing and observed.
The Missing Data Mechanisms
Missing Completely at Random, MCAR, means there is no relationship between (more…)
Two methods for dealing with missing data, vast improvements over traditional approaches, have become available in mainstream statistical software in the last few years.
Both of the methods discussed here require that the data are missing at random–not related to the missing values. If this assumption holds, resulting estimates (i.e., regression coefficients and standard errors) will be unbiased with no loss of power.
The first method is Multiple Imputation (MI). Just like the old-fashioned imputation (more…)
Q: Do most high impact journals require authors to state which method has been used on missing data?
I don’t usually get far enough in the publishing process to read journal requirements.
But based on my conversations with researchers who both review articles for journals and who deal with reviewers’ comments, I can offer this response.
I would be shocked if journal editors at top journals didn’t want information about the missing data technique. If you leave it out, they’ll either assume you didn’t have missing data or are using defaults like listwise deletion. (more…)
This year I hired a Quickbooks consultant to bring my bookkeeping up from the stone age. (I had been using Excel).
She had asked for some documents with detailed data, and I tried to send her something else as a shortcut. I thought it was detailed enough. It wasn’t, so she just fudged it. The bottom line was all correct, but the data that put it together was all wrong.
I hit the roof.Internally, only—I realized it was my own fault for not giving her the info she needed. She did a fabulous job.
But I could not leave the data fudged, even if it all added up to the right amount, and already reconciled. I had to go in and spend hours fixing it. Truthfully, I was a bit of a compulsive nut about it.
And then I had to ask myself why I was so uptight—if accountants think the details aren’t important, why do I? Statisticians are all about approximations and accountants are exact, right?
As it turns out, not so much.
But I realized I’ve had 20 years of training about the importance of data integrity. Sure, the results might be inexact, the analysis, the estimates, the conclusions. But not the data. The data must be clean.
Sparkling, if possible.
In research, it’s okay if the bottom line is an approximation. Because we’re never really measuring the whole population. And we can’t always measure precisely what we want to measure. But in the long run, it all averages out.
But only if the measurements we do have are as accurate as they possibly can be.