When we think about model assumptions, we tend to focus on assumptions like independence, normality, and constant variance. The other big assumption, which is harder to see or test, is that there is no specification error. The assumption of linearity is part of this, but it’s actually a bigger assumption.
What is this assumption of no specification error? (more…)
Transformations don’t always help, but when they do, they can improve your linear regression model in several ways simultaneously.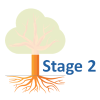
They can help you better meet the linear regression assumptions of normality and homoscedascity (i.e., equal variances). They also can help avoid some of the artifacts caused by boundary limits in your dependent variable — and sometimes even remove a difficult-to-interpret interaction.
(more…)
Why is it we can model non-linear effects in linear regression?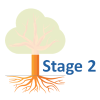
What the heck does it mean for a model to be “linear in the parameters?” (more…)
Should you drop outliers? Outliers are one of those statistical issues that everyone knows about, but most people aren’t sure how to deal with. Most parametric statistics, like means, standard deviations, and correlations, and every statistic based on these, are highly sensitive to outliers.
And since the assumptions of common statistical procedures, like linear regression and ANOVA, are also based on these statistics, outliers can really mess up your analysis.
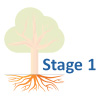
Despite all this, as much as you’d like to, it is NOT acceptable to
(more…)