In this webinar, we’ll discuss when tables and graphs are (and are not) appropriate and how people engage with each of these media.
Then we’ll discuss design principles for good tables and graphs and review examples that meet these principles. Finally, we’ll show that the choice between tables and graphs is not always dichotomous: tables can be incorporated into graphs and vice versa.
Participants will learn how to bring more thoughtfulness to the process of deciding when to use tables and when to use graphs in their work. They will also learn about design principles and examples they can adopt to create better tables and graphs.
Note: This training is an exclusive benefit to members of the Statistically Speaking Membership Program and part of the Stat’s Amore Trainings Series. Each Stat’s Amore Training is approximately 90 minutes long.
(more…)
Survival data models provide interpretation of data representing the time until an event occurs. In many situations, the event is death, but it can also represent the time to other bad events such as
cancer relapse or failure of a medical device. It can also be used to denote time to positive events such as pregnancy. Often patients are lost to follow-up prior to death, but you can still use the information about them while they were in your study to better estimate the survival probability over time.
This is done using the Kaplan-Meier curve, an approach developed by (more…)
In many fields, the only way to measure a construct of interest is to have someone produce ratings: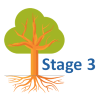
- radiologists’ ratings of disease presence or absence on an X-ray
- researchers rate the amount of bullying occurring in an observed classroom
- coders sort qualitative responses into different response categories
It’s well established in research that multiple raters need to rate the same stimuli to ensure ratings are accurate. There are a number of ways to measure the agreement among raters using measures of reliability. These differ depending on a host of details, including: the number of raters; whether ratings are nominal, ordinal, or numerical; and whether one rating can be considered a “Gold Standard.”
In this webinar, we will discuss these and other issues in measures of inter and intra rater reliability, the many variations of the Kappa statistic, and Intraclass correlations.
Note: This training is an exclusive benefit to members of the Statistically Speaking Membership Program and part of the Stat’s Amore Trainings Series. Each Stat’s Amore Training is approximately 90 minutes long.
About the Instructor
Audrey Schnell is a statistical consultant and trainer at The Analysis Factor.
Audrey first realized her love for research and, in particular, data analysis in a career move from clinical psychology to research in dementia. As the field of genetic epidemiology and statistical genetics blossomed, Audrey moved into this emerging field and analyzed data on a wide variety of common diseases believed to have a strong genetic component including hypertension, diabetes and psychiatric disorders. She helped develop software to analyze genetic data and taught classes in the US and Europe.
Audrey has worked for Case Western Reserve University, Cedars-Sinai, University of California at San Francisco and Johns Hopkins. Audrey has a Master’s Degree in Clinical Psychology and a Ph.D. in Epidemiology and Biostatistics.
Not a Member Yet?
It’s never too early to set yourself up for successful analysis with support and training from expert statisticians.
Just head over and sign up for Statistically Speaking.
You'll get access to this training webinar, 130+ other stats trainings, a pathway to work through the trainings that you need — plus the expert guidance you need to build statistical skill with live Q&A sessions and an ask-a-mentor forum.
This webinar, presented by Yasamin Miller, will cover broadly survey design and planning.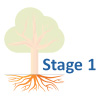
It will outline the advantages and disadvantages of the various data collection modes, types of samples available to target your population, how to obtain a representative sample, and how to avoid the pitfalls of bad questionnaire design.
Note: This training is an exclusive benefit to members of the Statistically Speaking Membership Program and part of the Stat’s Amore Trainings Series. Each Stat’s Amore Training is approximately 90 minutes long.
(more…)
There are many types and examples of ordinal variables: percentiles, ranks, likert scale items, to name a few.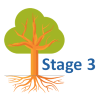
These are especially hard to know how to analyze–some people treat them as numerical, others emphatically say not to. Everyone agrees nonparametric tests work, but these are limited to testing only simple hypotheses and designs. So what do you do if you want to test something more elaborate?
In this webinar we’re going to lay out all the options and when each is (more…)
So you can’t randomize people into THAT condition? Now what?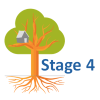
Let’s say you’re investigating the impact of smoking on social outcomes like depression, poverty, or quality of life. Your IRB, with good reason, won’t allow random assignment of smoking status to your participants.
But how can you begin to overcome the self selected nature of smoking among the study participants? What if self-selection is driving differences in outcomes? Well, one way is to use propensity score matching and analysis as a framework for your investigation.
The propensity score is the probability of group assignment conditional on observed baseline characteristics. In this way, the propensity score is a balancing score: conditional on the propensity score, the distribution of observed baseline covariates will be similar between treated and untreated subjects.
In this webinar, we’ll describe broadly what this method is and discuss different matching methods that can be used to create balanced samples of “treated” and “non-treated” participants. Finally, we’ll discuss some specific software resources that can be found to perform these analyses.
Note: This training is an exclusive benefit to members of the Statistically Speaking Membership Program and part of the Stat’s Amore Trainings Series. Each Stat’s Amore Training is approximately 90 minutes long.
(more…)