If you’ve been doing data analysis for very long, you’ve certainly come across terms, concepts, and processes of matrix algebra. Not just matrices, but: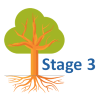
- Matrix addition and multiplication
- Traces and determinants
- Eigenvalues and Eigenvectors
- Inverting and transposing
- Positive and negative definite
(more…)
What’s the difference between Mixed and Multilevel Models? What about Hierarchical Models or Random Effects models?
I get this question a lot.
The answer: very little.
(more…)
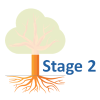
What are goodness of fit statistics? Is the definition the same for all types of statistical model? Do we run the same tests for all types of statistic model?
(more…)
Multilevel models and Mixed Models are generally the same thing. In our recent webinar on the basics of mixed
models, Random Intercept and Random Slope Models, we had a number of questions about terminology that I’m going to answer here.
If you want to see the full recording of the webinar, get it here. It’s free.
Q: Is this different from multi-level modeling?
A: No. I don’t really know the history of why we have the different names, but the difference in multilevel modeling (more…)
Mixed models are hard.
They’re abstract, they’re a little weird, and there is not a common vocabulary or notation for them.
But they’re also extremely important to understand because many data sets require their use.
Repeated measures ANOVA has too many limitations. It just doesn’t cut it any more.
One of the most difficult parts of fitting mixed models is figuring out which random effects to include in a model. And that’s hard to do if you don’t really understand what a random effect is or how it differs from a fixed effect. (more…)