Generalized linear mixed models (GLMMs) are incredibly useful—but they’re also a hard nut to crack.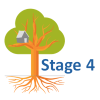
As an extension of generalized linear models, GLMMs include both fixed and random effects. They are particularly useful when an outcome variable and a set of predictor variables are measured repeatedly over time and the outcome variable is a binary, nominal, ordinal or count variable. These models accommodate nesting of subjects in higher level units such as schools, hospitals, etc., and can also incorporate predictor variables collected at these higher levels.
In this webinar, we’ll provide a gentle introduction to GLMMs, discussing issues like: (more…)
When you have data measuring the time to an event, you can examine the relationship between various predictor variables and the time to the event using a Cox proportional hazards model.
In this webinar, you will see what a hazard function is and describe the interpretations of increasing, decreasing, and constant hazard. Then you will examine the log rank test, a simple test closely tied to the Kaplan-Meier curve, and the Cox proportional hazards model.
Note: This training is an exclusive benefit to members of the Statistically Speaking Membership Program and part of the Stat’s Amore Trainings Series. Each Stat’s Amore Training is approximately 90 minutes long.
(more…)
Despite modern concerns about how to handle big data, there persists an age-old question: What can we do with small samples?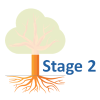
Sometimes small sample sizes are planned and expected. Sometimes not. For example, the cost, ethical, and logistical realities of animal experiments often lead to samples of fewer than 10 animals.
Other times, a solid sample size is intended based on a priori power calculations. Yet recruitment difficulties or logistical problems lead to a much smaller sample. In this webinar, we will discuss methods for analyzing small samples. Special focus will be on the case of unplanned small sample sizes and the issues and strategies to consider.
Note: This training is an exclusive benefit to members of the Statistically Speaking Membership Program and part of the Stat’s Amore Trainings Series. Each Stat’s Amore Training is approximately 90 minutes long.
(more…)
Statistically speaking, when we see a continuous outcome variable we often worry about outliers and how these extreme observations can impact our model.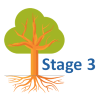
But have you ever had an outcome variable with no outliers because there was a boundary value at which accurate measurements couldn’t be or weren’t recorded?
Examples include:
- Income data where all values above $100,000 are recorded as $100k or greater
- Soil toxicity ratings where the device cannot measure values below 1 ppm
- Number of arrests where there are no zeros because the data set came from police records where all participants had at least one arrest
These are all examples of data that are truncated or censored. Failing to incorporate the truncation or censoring will result in biased results.
This webinar will discuss what truncated and censored data are and how to identify them.
There are several different models that are used with this type of data. We will go over each model and discuss which type of data is appropriate for each model.
We will then compare the results of models that account for truncated or censored data to those that do not. From this you will see what possible impact the wrong model choice has on the results.
Note: This training is an exclusive benefit to members of the Statistically Speaking Membership Program and part of the Stat’s Amore Trainings Series. Each Stat’s Amore Training is approximately 90 minutes long.
(more…)
A common situation with count outcome variables is there are a lot of zero values. The Poisson distribution used for modeling count variables takes into account that zeros are often the most
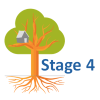
common value, but sometimes there are even more zeros than the Poisson distribution can account for.
This can happen in continuous variables as well–most of the distribution follows a beautiful normal distribution, except for the big stack of zeros.
This webinar will explore two ways of modeling zero-inflated data: the Zero Inflated model and the Hurdle model. Both assume there are two different processes: one that affects the probability of a zero and one that affects the actual values, and both allow different sets of predictors for each process.
We’ll explore these models as well as some related models, like Zero-One Inflated Beta models for proportion data.
Note: This training is an exclusive benefit to members of the Statistically Speaking Membership Program and part of the Stat’s Amore Trainings Series. Each Stat’s Amore Training is approximately 90 minutes long.
(more…)
In this webinar, we’ll discuss when tables and graphs are (and are not) appropriate and how people engage with each of these media.
Then we’ll discuss design principles for good tables and graphs and review examples that meet these principles. Finally, we’ll show that the choice between tables and graphs is not always dichotomous: tables can be incorporated into graphs and vice versa.
Participants will learn how to bring more thoughtfulness to the process of deciding when to use tables and when to use graphs in their work. They will also learn about design principles and examples they can adopt to create better tables and graphs.
Note: This training is an exclusive benefit to members of the Statistically Speaking Membership Program and part of the Stat’s Amore Trainings Series. Each Stat’s Amore Training is approximately 90 minutes long.
(more…)