In this video I will answer a question from a recent webinar Random Intercept and Random Slope Models.
We are answering questions here because we had over 500 people live on the webinar so we didn’t have time to get through all the questions.
If you missed the webinar live, this and the other questions in this series may make more sense if you watch that first. It was part of our free webinar series, The Craft of Statistical Analysis, and you can sign up to get the free recording, handout, and data set at this link:
http://TheCraftofStatisticalAnalysis.com/random-intercept-random-slope-models
Pretty much all of the common statistical models we use, with the exception of OLS Linear Models, use Maximum Likelihood estimation.
This includes favorites like:
That’s a lot of models.
If you’ve ever learned any of these, you’ve heard that some of the statistics that compare model fit in competing models require (more…)
In the past few months, I’ve gotten the same question from a few clients about using linear mixed models for repeated measures data. They want to take advantage of its ability to give unbiased results in the presence of missing data. In each case the study has two groups complete a pre-test and a post-test measure. Both of these have a lot of missing data.
The research question is whether the groups have different improvements in the dependent variable from pre to post test.
As a typical example, say you have a study with 160 participants.
90 of them completed both the pre and the post test.
Another 48 completed only the pretest and 22 completed only the post-test.
Repeated Measures ANOVA will deal with the missing data through listwise deletion. That means keeping only the 90 people with complete data. This causes problems with both power and bias, but bias is the bigger issue.
Another alternative is to use a Linear Mixed Model, which will use the full data set. This is an advantage, but it’s not as big of an advantage in this design as in other studies.
The mixed model will retain the 70 people who have data for only one time point. It will use the 48 people with pretest-only data along with the 90 people with full data to estimate the pretest mean.
Likewise, it will use the 22 people with posttest-only data along with the 90 people with full data to estimate the post-test mean.
If the data are missing at random, this will give you unbiased estimates of each of these means.
But most of the time in Pre-Post studies, the interest is in the change from pre to post across groups.
The difference in means from pre to post will be calculated based on the estimates at each time point. But the degrees of freedom for the difference will be based only on the number of subjects who have data at both time points.
So with only two time points, if the people with one time point are no different from those with full data (creating no bias), you’re not gaining anything by keeping those 72 people in the analysis.
Compare this to a study I also saw in consulting with 5 time points. Nearly all the participants had 4 out of the 5 observations. The missing data was pretty random–some participants missed time 1, others, time 4, etc. Only 6 people out of 150 had full data. Listwise deletion created a nightmare, leaving only 6 people in the data set.
Each person contributed data to 4 means, so each mean had a pretty reasonable sample size. Since the missingness was random, each mean was unbiased. Each subject fully contributed data and df to many of the mean comparisons.
With more than 2 time points and data that are missing at random, each subject can contribute to some change measurements. Keep that in mind the next time you design a study.
One of the most confusing things about mixed models arises from the way it’s coded in most statistical software. Of the ones I’ve used, only HLM sets it up differently and so this doesn’t apply.
But for the rest of them—SPSS, SAS, R’s lme and lmer, and Stata, the basic syntax requires the same pieces of information.
1. The dependent variable
2. The predictor variables for which to calculate fixed effects and whether those (more…)
I have recently worked with two clients who were running generalized linear mixed models in SPSS.
Both had repeated measures experiments with a binary outcome.
The details of the designs were quite different, of course. But both had pretty complicated combinations of within-subjects factors.
Fortunately, both clients are intelligent, have a good background in statistical modeling, and are willing to do the work to learn how to do this. So in both cases, we made a lot of progress in just a couple meetings.
I found it interesting, through, that both were getting stuck on the same subtle point. It’s the same point I was missing for a long time in my own learning of mixed models.
Once I finally got it, a huge light bulb turned on. (more…)
Repeated measures ANOVA is the approach most of us learned in stats classes for repeated measures and longitudinal data. It works very well in certain designs.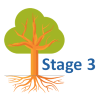
But it’s limited in what it can do. Sometimes trying to fit a data set into a repeated measures ANOVA requires too much data gymnastics. (more…)