When you need to compare a numeric outcome for two groups, what analysis do you think of first? Chances are, it’s the independent samples t-test. But that’s not the only, or always, the best option. In many situations, the Mann-Whitney U test is a better option.
The non-parametric Mann-Whitney U test is also called the Mann-Whitney-Wilcoxon test, or the Wilcoxon rank sum test. Non-parametric means that the hypothesis it’s testing is not about the parameter of a particular distribution.
It is part of a subgroup of non-parametric tests that are rank based. That means that the specific values of the outcomes are not important, only their order. In other words, we will be ranking the outcomes.
Like the t-test, this analysis tests whether two independent groups have similar typical outcomes. You can use it with numeric data, but unlike the t-test, it also works with ordinal data. Like the t-test, it is designed for comparisons, and not for estimation or prediction.
The biggest difference from the t-test is that it does not compare means. The Mann-Whitney U test determines whether a random observation from one group tends to be higher (or lower) than a random observation from the other group. Imagine choosing two observations, one from each group, over and over again. This test will determine whether one group is more likely to have the higher values.
It has many advantages: It is a straightforward comparison of means. There are versions for similar and different variances in the two groups. Many people are familiar with it.
(more…)
Spoiler alert, real data are seldom normally distributed. How does the population distribution influence the estimate of the population mean and its confidence interval?
To figure this out, we randomly draw 100 observations 100 times from three distinct populations and plot the mean and corresponding 95% confidence interval of each sample.
(more…)
by Jeff Meyer, MBA, MPA
One of the most important concepts in data analysis is that the analysis needs to be appropriate for the scale of measurement of the variable. The focus of these decisions about scale tends to focus on levels of measurement: nominal, ordinal, interval, ratio.
These levels of measurement tell you about the amount of information in the variable. But there are other ways of distinguishing the scales that are also important and often overlooked.
(more…)
Most of us know that binary logistic regression is appropriate when the outcome variable has two possible outcomes: success and failure.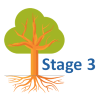
There are two more situations that are also appropriate for binary logistic regression, but they don’t always look like they should be.
(more…)
The normal distribution is so ubiquitous in statistics that those of us who use a lot of statistics tend to forget it’s not always so common in actual data.
And since the normal distribution is continuous, many people describe all numerical variables as continuous. I get it: I’m guilty of using those terms interchangeably, too, but they’re not exactly the same.
Numerical variables can be either continuous or discrete.
The difference? Continuous variables can take any number within a range. Discrete variables can only be whole numbers.
So 3.04873658 is a possible value of a continuous variable, but not discrete.
Count variables, as the name implies, are frequencies of some event or state. Number of arrests, fish (more…)
Last month I did a webinar on Poisson and negative binomial models for count data. With a few hundred participants, we ran out of time to get through all the questions, so I’m answering some of them here on the blog.
This set of questions are all related to when it’s appropriate to treat count data as continuous and run the more familiar and simpler linear model.
Q: Do you have any guidelines or rules of thumb as far as how many discrete values an outcome variable can take on before it makes more sense to just treat it as continuous?
The issue usually isn’t a matter of how many values there are.
(more…)