Linear regression with a continuous predictor is set up to measure the constant relationship between that predictor and a continuous outcome.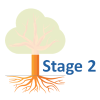
This relationship is measured in the expected change in the outcome for each one-unit change in the predictor.
One big assumption in this kind of model, though, is that this rate of change is the same for every value of the predictor. It’s an assumption we need to question, though, because it’s not a good approach for a lot of relationships.
Segmented regression allows you to generate different slopes and/or intercepts for different segments of values of the continuous predictor. This can provide you with a wealth of information that a non-segmented regression cannot.
In this webinar, we will cover (more…)
There are many rules of thumb in statistical analysis that make decision making and understanding results much easier.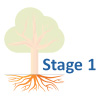
Have you ever stopped to wonder where these rules came from, let alone if there is any scientific basis for them? Is there logic behind these rules, or is it propagation of urban legends?
In this webinar, we’ll explore and question the origins, justifications, and some of the most common rules of thumb in statistical analysis, like:
(more…)
There are two main types of factor analysis: exploratory and confirmatory. Exploratory factor analysis (EFA) is data driven, such that the collected data determines the resulting factors.
Confirmatory factor analysis (CFA) is used to test factors that have been developed a priori.
Think of CFA as a process for testing what you already think you know.
CFA is an integral part of structural equation modeling (SEM) and path analysis. The hypothesized factors should always be validated with CFA in a measurement model prior to incorporating them into a path or structural model. Because… garbage in, garbage out.
CFA is also a useful tool in checking the reliability of a measurement tool with a new population of subjects, or to further refine an instrument which is already in use.
Elaine will provide an overview of CFA. She will also (more…)
One of the biggest challenges that data analysts face is communicating statistical results to our clients, advisors, and colleagues who don’t have a statistics background.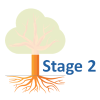
Unfortunately, the way that we learn statistics is not usually the best way to communicate our work to others, and many of us are left on our own to navigate what is arguably the most important part of our work.
In this webinar, we will cover how to: (more…)
Generalized linear mixed models (GLMMs) are incredibly useful tools for working with complex, multi-layered data. But they can be tough to master.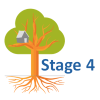
In this follow-up to October’s webinar (“A Gentle Introduction to Generalized Linear Mixed Models – Part 1”), we’ll cover important topics like:
– Distinction between crossed and nested grouping factors
– Software choices for implementation of GLMMs (more…)
The LASSO model (Least Absolute Shrinkage and Selection Operator) is a recent development that allows you to find a good fitting model in the regression context. It avoids many of the problems
of overfitting that plague other model-building approaches.
In this Statistically Speaking Training, guest instructor Steve Simon, PhD, explains what overfitting is — and why it’s a problem.
Then he illustrates the geometry of the LASSO model in comparison to other regression approaches, ridge regression and stepwise variable selection.
Finally, he shows you how LASSO regression works with a real data set.
Note: This training is an exclusive benefit to members of the Statistically Speaking Membership Program and part of the Stat’s Amore Trainings Series. Each Stat’s Amore Training is approximately 90 minutes long.
(more…)