The first real data set I ever analyzed was from my senior honors thesis as an undergraduate psychology major. I had taken both intro stats and an ANOVA class, and I applied all my new skills with gusto, analyzing every which way.
It wasn’t too many years into graduate school that I realized that these data analyses were a bit haphazard. (Okay, a LOT). And honestly, not at all well thought out.
A few decades of data analysis experience later, I realized that’s just a symptom of being an inexperienced data analyst.
But even experienced data analysts can get off track. It’s especially easy with large data sets with many variables. It’s just so tempting to try one thing, then another, and pretty soon you’ve spent weeks getting nowhere.
(more…)
There are many designs that could be considered Repeated Measures design, and they all have one key feature: you measure the outcome variable
for each subject on several occasions, treatments, or locations.
Understanding this design is important for avoiding analysis mistakes. For example, you can’t treat multiple observations on the same subject as independent observations.
Example
Suppose that you recruit 10 subjects (more…)
It’s easy to make things complex without meaning to. Especially in statistical analysis.
Sometimes that complexity is unavoidable. You have ethical and practical constraints on your study design and variable measurement. Or the data just don’t behave as you expected. Or the only research question of interest is one that demands many variables.
But sometimes it isn’t. Seemingly innocuous decisions lead to complicated analyses. These decisions occur early in the design, research questions, or variable choice.
(more…)
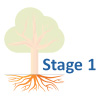
One component often overlooked in the ‘Define & Design’ phase of a study, is writing the analysis plan. The statistical analysis plan integrates a lot of information about the study including the research question, study design, variables and data used, and the type of statistical analysis that will be conducted.
(more…)
by Danielle Bodicoat
Statistics can tell us a lot about our data, but it’s also important to consider where the underlying data came from when interpreting results, whether they’re our own or somebody else’s.
Not all evidence is created equally, and we should place more trust in some types of evidence than others.
(more…)
One activity in data analysis that can seem impossible is the quest to find the right analysis. I applaud the conscientiousness and integrity that
underlies this quest.
The problem: in many data situations there isn’t one right analysis.
(more…)