Are you learning Multilevel Models? Do you feel ready? Or in over your head?
It’s a very common analysis to need to use. I have to say, learning it is not so easy on your own. The concepts of random effects are hard to wrap your head around and there is a ton of new vocabulary and notation. Sadly, this vocabulary and notation is not consistent across articles, books, and software, so you end up having to do a lot of translating.
But the stronger your foundation in linear regression, ANOVA, and how they fit together, the better equipped you’ll be to take this on.
Why? Because multilevel models are just general linear models with extra sources of variation. Learning multilevel models is much harder if you’re still unsure about some of the concepts in linear regression. You want to focus on figuring out what a random slope really means, not a centered predictor.
So here are four concepts in linear regression that you really, really should get clear about before you attempt to learn multilevel models. It won’t make it easy, but it will make it easier.
1. What centering does to your coefficients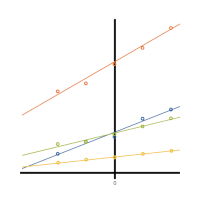
Intercepts are important in multilevel models.
And centering affects intercepts. Both fixed and random intercepts. So you have to be careful about making sure you’ve centered variables that will give you interpretable random intercepts. The cool part is you can often make strategic decisions about how to center each numerical predictor to make intercepts meaningful.
Interactions are important also in multilevel models. Random slopes are, at their heart, interactions.
And guess what? Centering a variable that’s in an interaction also affects coefficients. So once again, you want to make sure you’re centering predictor variables with intention.
2. Working with both categorical and continuous predictors and interpreting their coefficients
Once you get into mixed models, there will be situations where it’s useful to use both dummy and effect coding. And even if you only ever use dummy coding, you need to be able to interpret their coefficients and know how they work.
Likewise, you want to be able to understand what it means if you make a discrete numerical predictor variable continuous or categorical. If that discrete predictor is truly numerical, you may be able to choose. Depending on the design, this choice can have a big impact on whether or not you can fit a random slope.
It also has a big impact on the information do you get from it and what does it means.
3. Interactions
I know you know that you have to leave in lower order terms for each interaction (right?). Make sure you can interpret interactions regardless of how many categorical and continuous variables they contain. And make sure you can interpret an interaction regardless of whether the variables in the interaction are both continuous, both categorical, or one of each.
4. Polynomial terms
Random slopes can be hard enough to grasp (and keep straight from random intercepts). Random curvature is worse. Know thy Polynomials.
There are a lot of situations in mixed models where you’re fitting trajectories for each subject or cluster. Especially in repeated measures or longitudinal data. It turns out mixed models are flexible enough to accommodate random quadratic terms to fit curves instead of lines for those trajectories.
It gives you a lot of flexibility, but it’s really hard to apply unless you know how to fit a curve within a linear regression. It’s actually not that hard, but learning it in the context of linear regression is much easier than once you get into random effects.
Fitting it all together
And finally, understand how they all fit together. These four concepts really come down to understanding what the estimates in your model mean. How to interpret them. How to choose which one to use to fit the data, the design, and to answer your research question.
And that will come largely from practice, asking questions, and relearning the basics in the context of your data.
Beyond Learning Multilevel Models
The big advantage is that understanding these concepts will behoove you not only in learning multilevel models, but in learning any advanced modeling. Having a solid understanding of the ins and outs of linear models will help you in any Stage 3 model–nonlinear models, logistic regression, Cox regression, and so on.
Hi Sam,
This is neither–just an article.
However, we do cover all of these things in the Interpreting (Even Tricky) Regression Coefficients Workshop. It’s actually one of the reasons I developed the workshop, is I saw people still struggling with these concepts as they were also trying to learn multilevel modeling.
This is the link, so you can get all the information about when we are next offering it.
http://www.theanalysisinstitute.com/workshops/IRC/index.html
Would this be a webinar or work shop? Cost? timing?
Regards,
Sam