the craft of statistical analysis free webinars
Fixed and Random Factors in Mixed Models
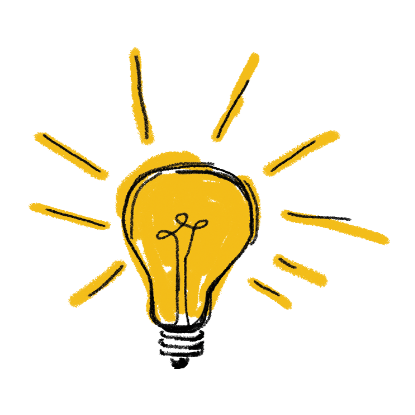
What is the difference between fixed and random factors in Mixed Models? How do you figure out which one you have? learn more
The Pathway: Steps for Staying Out of the
Weeds in Any Data Analysis
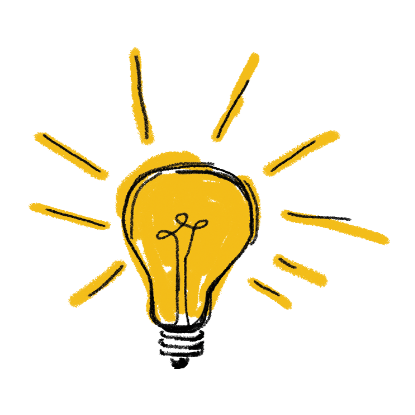
Ever find yourself redoing parts of your data analysis? Getting so lost in the weeds that you’re not sure about your next step? Or trying every possible option and suddenly the whole day (or week) is gone? learn more
Effect Size Statistics
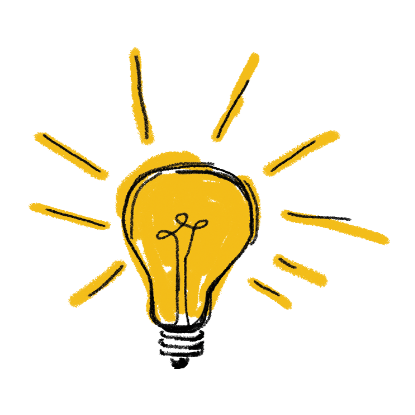
Effect Size Statistics are all the rage. Journal editors want to see them in every results section.
You need them for performing sample size estimates (And editors want those too). But statistical software doesn’t always give you the effect sizes you need… learn more
statistically speaking member trainings
Interactions in ANOVA and
Regression Models, Part 1
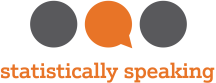
There is something about interactions that is incredibly confusing. An interaction between two predictor variables means that one predictor variable affects a third variable differently at different values of the other predictor. How you understand that interaction depends on many things. learn more
MANOVA
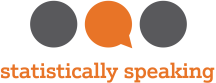
MANOVA is the multivariate (meaning multiple dependent variables) version of ANOVA, but there are many misconceptions about it. learn more
Multiple Comparisons
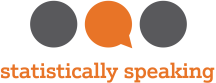
Whenever you run multiple statistical tests on the same set of data, you run into the problem of the Familywise Error Rate. This is a complicated and controversial issue in statistics — even statisticians argue about whether it’s a problem, when it’s a problem, and what to do about it. learn more
Marginal Means, Your New Best Friend
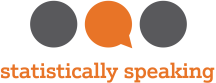
Interpreting regression coefficients can be tricky, especially when the model has interactions or categorical predictors (or worse – both). But there is a secret weapon that can help you make sense of your regression results: marginal means. learn more
Crossed and Nested Factors
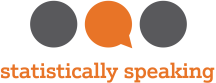
We often talk about nested factors in mixed models — students nested in classes, observations nested within subject. But in all but the simplest designs, it’s not that straightforward. learn more
An Overview of Effect Size Statistics and
Why They are So Important
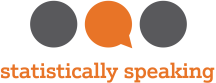
Whenever we run an analysis of variance or run a regression one of the first things we do is look at the p-value of our predictor variables to determine whether they are statistically significant. When the variable is statistically significant, did you ever stop and ask yourself how significant it is? How large of an impact does it actually have on our outcome variable? learn more
ANCOVA (Analysis of Covariance)
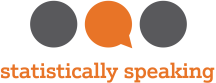
Analysis of Covariance (ANCOVA) is a type of linear model that combines the best abilities of linear regression with the best of Analysis of Variance. It allows you to test differences in group means and interactions, just like ANOVA, while covarying out the effect of a continuous covariate. learn more
articles at the analysis factor
An Example of Specifying Within-Subjects
Factors in Repeated Measures
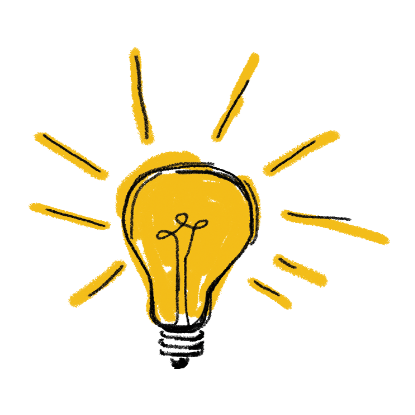
Some repeated measures designs make it quite challenging to specify within-subjects factors. Especially difficult is when the design contains two “levels” of repeat, but your interest is in testing just one. learn more
When Main Effects are Not Significant,
But the Interaction Is
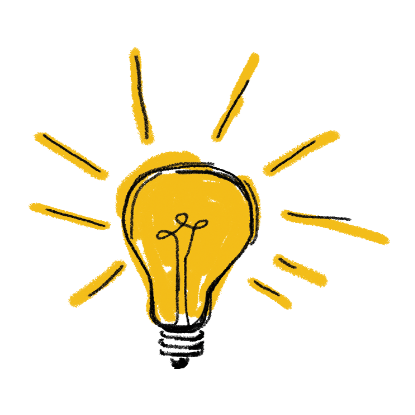
If you have significant a significant interaction effect and non-significant main effects, would you interpret the interaction effect? It’s a question I get pretty often, and it’s a more straightforward answer than most. learn more
Actually, You Can Interpret Some Main Effects
In the Presence of an Interaction
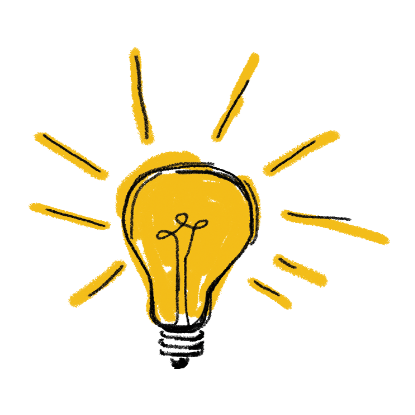
One of those “rules” about statistics you often hear is that you can’t interpret a main effect in the presence of an interaction. Stats professors seem particularly good at drilling this into students’ brains. Unfortunately, it’s not true. At least not always. learn more
When Does Repeated Measures ANOVA
Not Work for Repeated Measures Data?
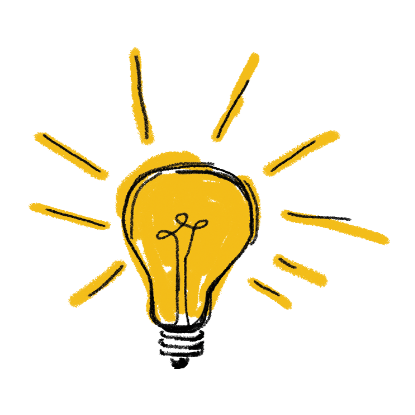
Repeated measures ANOVA is the approach most of us learned in stats classes for repeated measures and longitudinal data. It works very well in certain designs. But it’s limited in what it can do. Sometimes trying to fit a data set into a repeated measures ANOVA requires too much data gymnastics. learn more
Non-parametric ANOVA in SPSS
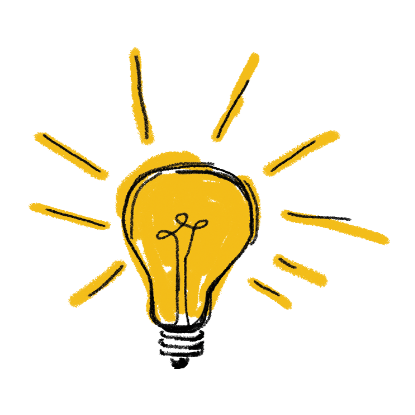
I sometimes get asked questions that many people need the answer to. Here’s one about non-parametric ANOVA in SPSS. learn more
When Assumptions of ANCOVA are Irrelevant
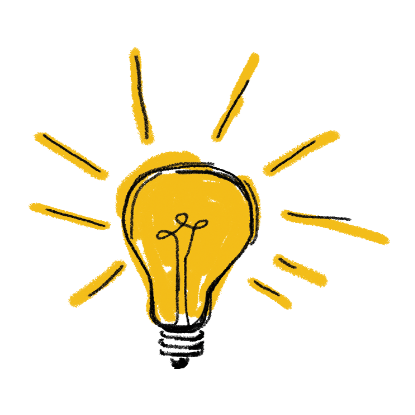
Every once in a while, I work with a client who is stuck between a particular statistical rock and hard place. It happens when they’re trying to run an analysis of covariance (ANCOVA) model because they have a categorical independent variable and a continuous covariate. learn more
ANCOVA Assumptions: When Slopes are Unequal
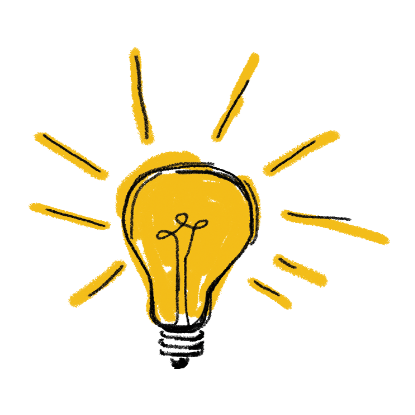
There are two oft-cited assumptions for Analysis of Covariance (ANCOVA), which is used to assess the effect of a categorical independent variable on a numerical dependent variable while controlling for a numerical covariate. 1, the independent variable and the covariate are independent of each other. And 2, there is no interaction between independent variable and the covariate. learn more
The Difference Between Crossed and Nested Factors
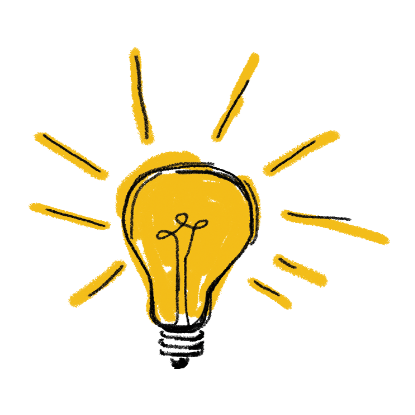
One of those tricky, but necessary, concepts in statistics is the difference between crossed and nested factors. As a reminder, a factor is any categorical independent variable. In experiments, or any randomized designs, these factors are often manipulated. Experimental manipulations (like Treatment vs. Control) are factors. learn more
Checking the Normality Assumption
For an ANOVA Model
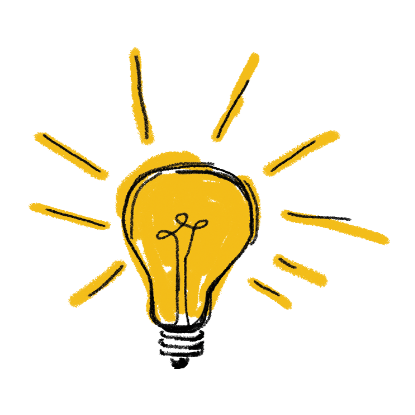
I received a great question from a past participant in my Assumptions of Linear Models workshop. It’s one of those quick questions without a quick answer. Or rather, without a quick and useful answer. learn more
A Comparison of Effect Size Statistics
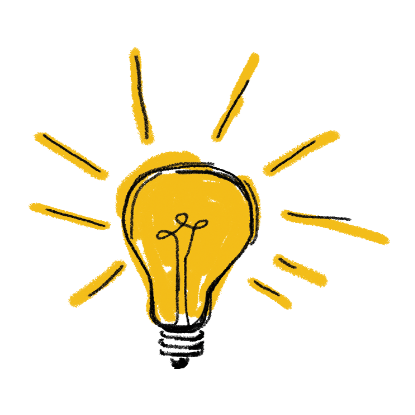
If you’re in a field that uses Analysis of Variance, you have surely heard that p-values alone don’t indicate the size of an effect. You also need to give some sort of effect size measure. Why? Because with a big enough sample size, any difference in means, no matter how small, can be statistically significant. learn more